引言
膨脹與腐蝕是圖像處理中兩種最基本的形態(tài)學(xué)操作,膨脹將目標(biāo)點(diǎn)融合到背景中,向外部擴(kuò)展,腐蝕與膨脹意義相反,消除連通的邊界,使邊界向內(nèi)收縮。在本文中我們將了解使用內(nèi)核的圖像膨脹與腐蝕的基本原理。
讓我們開始吧,同樣我們需要導(dǎo)入必需的庫(kù)。
import numpy as np
import matplotlib.pyplot as plt
from skimage.io import imread, imshow
from skimage.draw import circle
from skimage.morphology import erosion, dilation
首先讓我們創(chuàng)建一個(gè)容易操作的形狀--一個(gè)簡(jiǎn)單的圓。
circ_image = np.zeros((100, 100))
circ_image[circle(50, 50, 25)] = 1
imshow(circ_image);
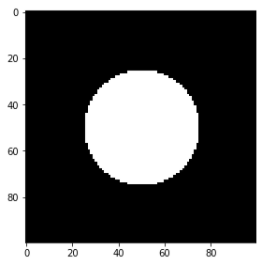
現(xiàn)在讓我們定義一個(gè)內(nèi)核。
cross = np.array([[0,1,0],
[1,1,1],
[0,1,0]])
imshow(cross, cmap = 'gray');
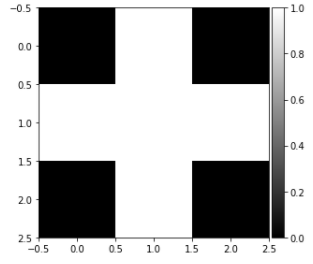
將腐蝕函數(shù)應(yīng)用到創(chuàng)建的圓上。
eroded_circle = erosion(circ_image, cross)
imshow(eroded_circle);
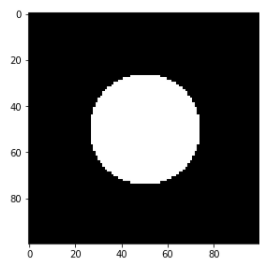
圖像看起來幾乎一模一樣。要看到那些微小的差異,我們必須仔細(xì)查看圖像。
linecolor = 'red'
fig, ax = plt.subplots(1, 2, figsize=(12, 5))
ax[0].imshow(circ_image, cmap = 'gray');
ax[0].set_title('Original', fontsize = 19)
ax[0].axvline(x = 25, color = linecolor)
ax[0].axvline(x = 75, color = linecolor)
ax[0].axhline(y = 25, color = linecolor)
ax[0].axhline(y = 75, color = linecolor)
ax[1].imshow(eroded_circle, cmap = 'gray');
ax[1].set_title('Eroded', fontsize = 19)
ax[1].axvline(x = 25, color = linecolor)
ax[1].axvline(x = 75, color = linecolor)
ax[1].axhline(y = 25, color = linecolor)
ax[1].axhline(y = 75, color = linecolor)
fig.tight_layout()
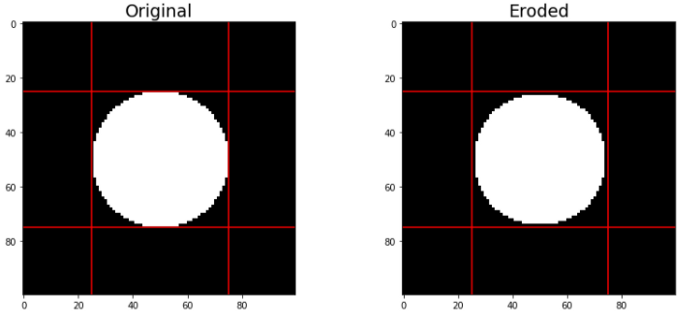
我們可以看到,被腐蝕的圓已經(jīng)略微縮小了。這就是腐蝕一個(gè)對(duì)象的意義。如果我們對(duì)腐蝕函數(shù)進(jìn)行迭代,它的效果會(huì)變得非常明顯。
def multi_erosion(image, kernel, iterations):
for i in range(iterations):
image = erosion(image, kernel)
return image
ites = [2,4,6,8,10,12,14,16,18,20]
fig, ax = plt.subplots(2, 5, figsize=(17, 5))
for n, ax in enumerate(ax.flatten()):
ax.set_title(f'Iterations : {ites[n]}', fontsize = 16)
new_circle = multi_erosion(circ_image, cross, ites[n])
ax.imshow(new_circle, cmap = 'gray');
ax.axis('off')
fig.tight_layout()
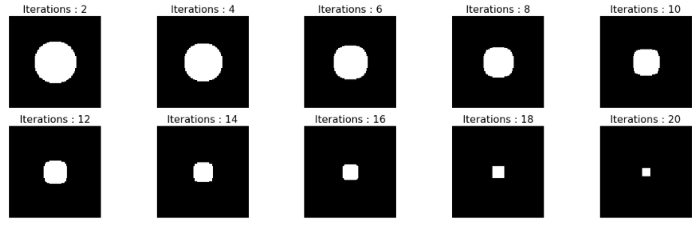
上圖清楚地顯示了圖像是如何被腐蝕的?,F(xiàn)在讓我們嘗試改變內(nèi)核,如果我們使用水平線和垂直線內(nèi)核代替交叉內(nèi)核會(huì)怎樣呢?
h_line = np.array([[0,0,0],
[1,1,1],
[0,0,0]])
v_line = np.array([[0,1,0],
[0,1,0],
[0,1,0]])
fig, ax = plt.subplots(1, 2, figsize=(15, 5))
ax[0].imshow(h_line, cmap='gray');
ax[1].imshow(v_line, cmap='gray');
fig.tight_layout()
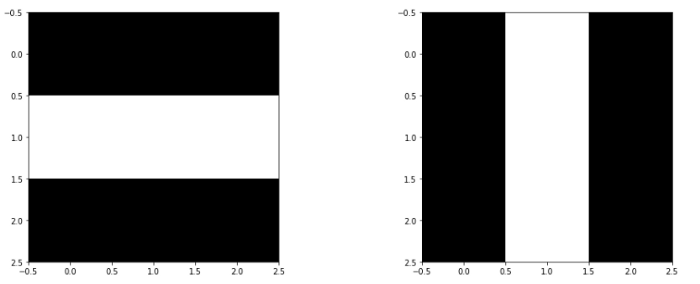
ites = [2,4,6,8,10,12,14,16,18,20]
fig, ax = plt.subplots(2, 5, figsize=(17, 5))
for n, ax in enumerate(ax.flatten()):
ax.set_title(f'Horizontal Iterations : {ites[n]}', fontsize = 12)
new_circle = multi_erosion(circ_image, h_line, ites[n])
ax.imshow(new_circle, cmap = 'gray');
ax.axis('off')
fig.tight_layout()
fig, ax = plt.subplots(2, 5, figsize=(17, 5))
for n, ax in enumerate(ax.flatten()):
ax.set_title(f'Vertical Iterationss : {ites[n]}', fontsize = 12)
new_circle = multi_erosion(circ_image, v_line, ites[n])
ax.imshow(new_circle, cmap = 'gray');
ax.axis('off')
fig.tight_layout()
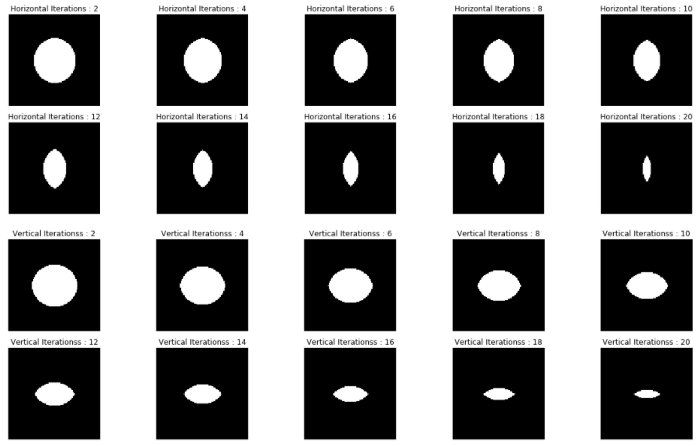
正如我們所看到的,水平和垂直的腐蝕以不同的方式影響著圖像。使用水平內(nèi)核我們得到一個(gè)垂直方向細(xì)長(zhǎng)的圓;而使用垂直內(nèi)核我們得到一個(gè)水平方向細(xì)長(zhǎng)的圓。
你可能會(huì)奇怪,為什么使用垂直內(nèi)核,會(huì)得到一個(gè)水平方向細(xì)長(zhǎng)的圓呢?
因?yàn)楦g函數(shù)是分別尋找垂直和水平的線條,并慢慢把它們削掉。膨脹函數(shù)將會(huì)讓我們更清晰的理解這一點(diǎn)。
使用下面的函數(shù)設(shè)置處理的圖像、膨脹內(nèi)核以及迭代次數(shù)。
def multi_dilation(image, kernel, iterations):
for i in range(iterations):
image = dilation(image, kernel)
return image
讓我們看一下處理后的圖像有什么不同。
dilated_circle = multi_dilation(circ_image, cross, 1)
linecolor = 'red'
fig, ax = plt.subplots(1, 2, figsize=(12, 5))
ax[0].imshow(circ_image, cmap = 'gray');
ax[0].set_title('Original', fontsize = 19)
ax[0].axvline(x = 25, color = linecolor)
ax[0].axvline(x = 75, color = linecolor)
ax[0].axhline(y = 25, color = linecolor)
ax[0].axhline(y = 75, color = linecolor)
ax[1].imshow(dilated_circle, cmap = 'gray');
ax[1].set_title('Dilated', fontsize = 19)
ax[1].axvline(x = 25, color = linecolor)
ax[1].axvline(x = 75, color = linecolor)
ax[1].axhline(y = 25, color = linecolor)
ax[1].axhline(y = 75, color = linecolor)
fig.tight_layout()
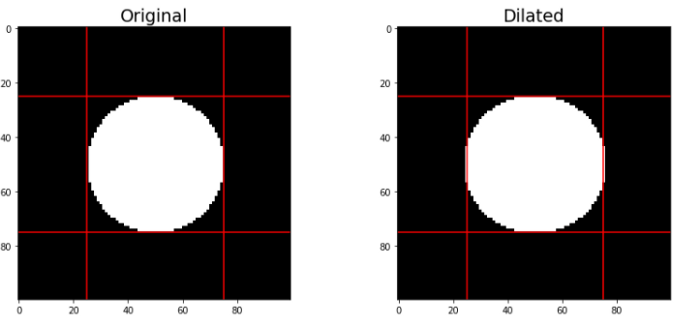
可以清楚地看到圓現(xiàn)在已經(jīng)越過了紅線,這清楚地表明它已經(jīng)擴(kuò)大了。現(xiàn)在讓我們對(duì)水平和垂直擴(kuò)張進(jìn)行迭代。
ites = [2,4,6,8,10,12,14,16,18,20]
fig, ax = plt.subplots(2, 5, figsize=(17, 5))
for n, ax in enumerate(ax.flatten()):
ax.set_title(f'Horizontal Iterations : {ites[n]}', fontsize =
12)
new_circle = multi_dilation(circ_image, h_line, ites[n])
ax.imshow(new_circle, cmap = 'gray');
ax.axis('off')
fig.tight_layout()
fig, ax = plt.subplots(2, 5, figsize=(17, 5))
for n, ax in enumerate(ax.flatten()):
ax.set_title(f'Vertical Iterationss : {ites[n]}', fontsize = 12)
new_circle = multi_dilation(circ_image, v_line, ites[n])
ax.imshow(new_circle, cmap = 'gray');
ax.axis('off')
fig.tight_layout()
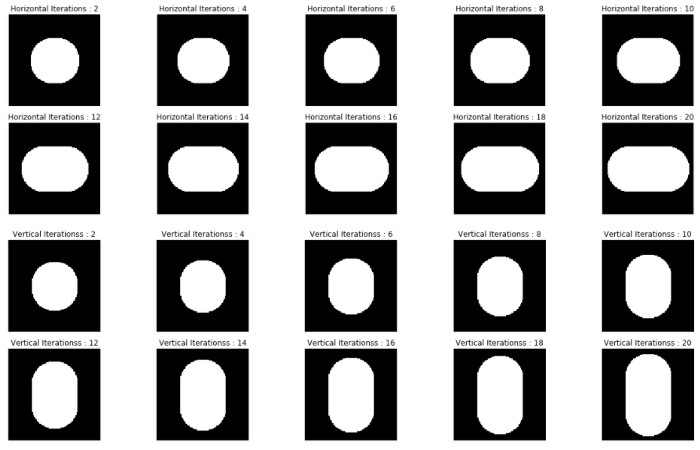
現(xiàn)在可以非常清楚地看到,水平擴(kuò)張?jiān)黾恿藞D像寬度,而垂直擴(kuò)張?jiān)黾恿藞D像高度。
現(xiàn)在我們已經(jīng)了解了膨脹與腐蝕的基本原理,下面來看一個(gè)相對(duì)復(fù)雜的圖像。
complex_image = imread('complex_image.png')
imshow(complex_image);
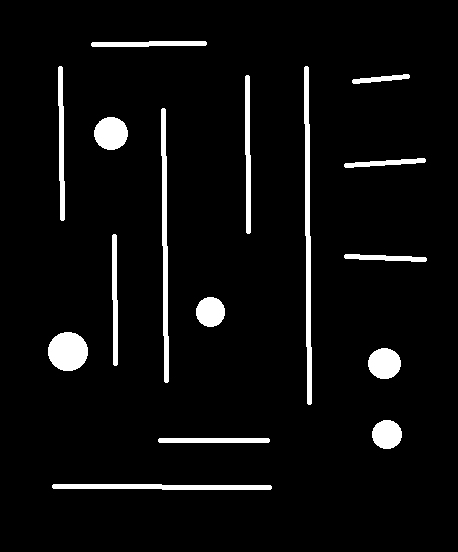
在上面的圖像中,我們看到了水平線、垂直線和圓的混合物。我們可以使用膨脹和腐蝕函數(shù)孤立地觀察每一種形狀。
為了得到圓,我們可以先腐蝕垂直的線,再腐蝕水平的線。但要記住最后要對(duì)圖像進(jìn)行膨脹,因?yàn)楦g函數(shù)同樣腐蝕了圓。
step_1 = multi_erosion(complex_image, h_line,3)
step_2 = multi_erosion(step_1, v_line,3)
step_3 = multi_dilation(step_2, h_line,3)
step_4 = multi_dilation(step_3, v_line,3)
steps = [step_1, step_2, step_3, step_4]
names = ['Step 1', 'Step 2', 'Step 3', 'Step 4']
fig, ax = plt.subplots(2, 2, figsize=(10, 10))
for n, ax in enumerate(ax.flatten()):
ax.set_title(f'{names[n]}', fontsize = 22)
ax.imshow(steps[n], cmap = 'gray');
ax.axis('off')
fig.tight_layout()
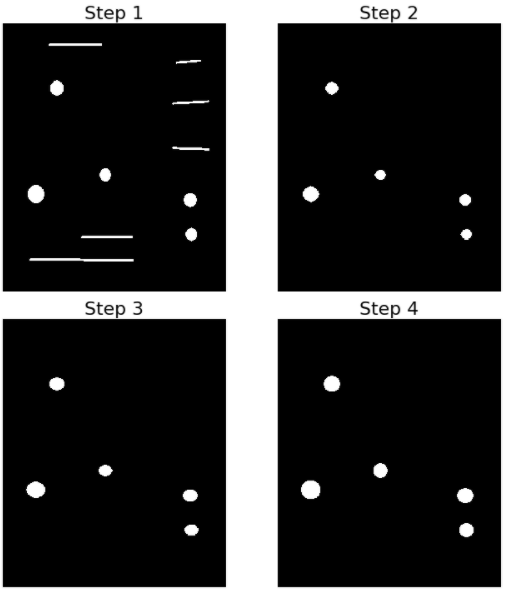
同樣,下面的代碼將得到水平的線。
step_1 = multi_erosion(complex_image, cross, 20)
step_2 = multi_dilation(step_1, h_line, 20)
step_3 = multi_dilation(step_2, v_line,2)
steps = [step_1, step_2, step_3]
names = ['Step 1', 'Step 2', 'Step 3']
fig, ax = plt.subplots(1, 3, figsize=(10, 10))
for n, ax in enumerate(ax.flatten()):
ax.set_title(f'{names[n]}', fontsize = 22)
ax.imshow(steps[n], cmap = 'gray');
ax.axis('off')
fig.tight_layout()

為了得到垂直的線,我們可以創(chuàng)建一個(gè)新的內(nèi)核。
long_v_line = np.array([[0,1,0],
[0,1,0],
[0,1,0],
[0,1,0],
[0,1,0]])
step_1 = multi_erosion(complex_image, long_v_line, 10)
step_2 = multi_dilation(step_1 ,long_v_line, 10)
steps = [step_1, step_2]
names = ['Step 1', 'Step 2']
fig, ax = plt.subplots(1, 2, figsize=(10, 10))
for n, ax in enumerate(ax.flatten()):
ax.set_title(f'{names[n]}', fontsize = 22)
ax.imshow(steps[n], cmap = 'gray');
ax.axis('off')
fig.tight_layout()
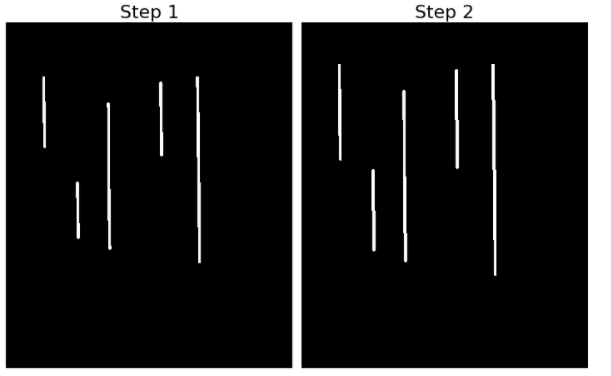
注意,內(nèi)核并不局限于本文中提到的這幾種,可以根據(jù)不同的需求自己定義合適的內(nèi)核。
總結(jié)
內(nèi)核腐蝕和膨脹是圖像處理領(lǐng)域需要理解的基本概念。它們甚至可能是任何圖像處理模塊的第一課。直觀地理解它們將是你以后在這個(gè)領(lǐng)域成功的關(guān)鍵。
到此這篇關(guān)于Python圖像處理之膨脹與腐蝕的操作的文章就介紹到這了,更多相關(guān)Python圖像膨脹與腐蝕內(nèi)容請(qǐng)搜索腳本之家以前的文章或繼續(xù)瀏覽下面的相關(guān)文章希望大家以后多多支持腳本之家!
您可能感興趣的文章:- 2021年最新用于圖像處理的Python庫(kù)總結(jié)
- python圖像處理基本操作總結(jié)(PIL庫(kù)、Matplotlib及Numpy)
- Python圖像處理之圖像拼接
- python數(shù)字圖像處理之估計(jì)噪聲參數(shù)
- python opencv圖像處理(素描、懷舊、光照、流年、濾鏡 原理及實(shí)現(xiàn))
- 基于python的opencv圖像處理實(shí)現(xiàn)對(duì)斑馬線的檢測(cè)示例
- Python圖像處理之圖片拼接和堆疊案例教程