我們在用神經(jīng)網(wǎng)絡(luò)求解PDE時, 經(jīng)常要用到輸出值對輸入變量(不是Weights和Biases)求導(dǎo); 在訓(xùn)練WGAN-GP 時, 也會用到網(wǎng)絡(luò)對輸入變量的求導(dǎo)。
以上兩種需求, 均可以用pytorch 中的autograd.grad() 函數(shù)實現(xiàn)。
autograd.grad(outputs, inputs, grad_outputs=None, retain_graph=None, create_graph=False, only_inputs=True, allow_unused=False)
outputs
: 求導(dǎo)的因變量(需要求導(dǎo)的函數(shù))
inputs
: 求導(dǎo)的自變量
grad_outputs
: 如果 outputs為標量,則grad_outputs=None,也就是說,可以不用寫; 如果outputs 是向量,則此參數(shù)必須寫,不寫將會報如下錯誤:

那么此參數(shù)究竟代表著什么呢?
先假設(shè)
為一維向量, 即可設(shè)自變量因變量分別為
, 其對應(yīng)的 Jacobi 矩陣為
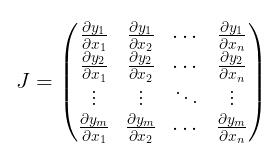
grad_outputs 是一個shape 與 outputs 一致的向量, 即

在給定grad_outputs 之后,真正返回的梯度為
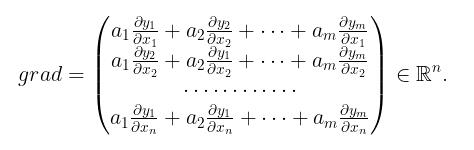
為方便下文敘述我們引入記號 
其次假設(shè)
,第i個列向量對應(yīng)的Jacobi矩陣為
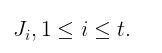
此時的grad_outputs 為(維度與outputs一致)

由第一種情況, 我們有
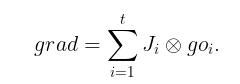
也就是說對輸出變量的列向量求導(dǎo),再經(jīng)過權(quán)重累加。
若
沿用第一種情況記號
, 其中每一個
均由第一種方法得出,
即對輸入變量列向量求導(dǎo),之后按照原先順序排列即可。
retain_graph: True 則保留計算圖, False則釋放計算圖
create_graph: 若要計算高階導(dǎo)數(shù),則必須選為True
allow_unused: 允許輸入變量不進入計算
下面我們看一下具體的例子:
import torch
from torch import autograd
x = torch.rand(3, 4)
x.requires_grad_()
觀察 x 為

不妨設(shè) y 是 x 所有元素的和, 因為 y是標量,故計算導(dǎo)數(shù)不需要設(shè)置grad_outputs
y = torch.sum(x)
grads = autograd.grad(outputs=y, inputs=x)[0]
print(grads)
結(jié)果為
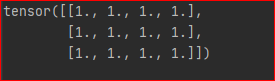
若y是向量
y = x[:,0] +x[:,1]
# 設(shè)置輸出權(quán)重為1
grad = autograd.grad(outputs=y, inputs=x, grad_outputs=torch.ones_like(y))[0]
print(grad)
# 設(shè)置輸出權(quán)重為0
grad = autograd.grad(outputs=y, inputs=x, grad_outputs=torch.zeros_like(y))[0]
print(grad)
結(jié)果為
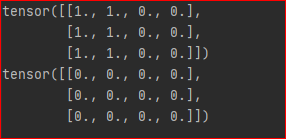
最后, 我們通過設(shè)置 create_graph=True 來計算二階導(dǎo)數(shù)
y = x ** 2
grad = autograd.grad(outputs=y, inputs=x, grad_outputs=torch.ones_like(y), create_graph=True)[0]
grad2 = autograd.grad(outputs=grad, inputs=x, grad_outputs=torch.ones_like(grad))[0]
print(grad2)
結(jié)果為
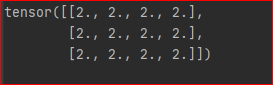
綜上,我們便搞清楚了它的求導(dǎo)機制。
補充:pytorch學(xué)習(xí)筆記:自動微分機制(backward、torch.autograd.grad)
一、前言
神經(jīng)網(wǎng)絡(luò)通常依賴反向傳播求梯度來更新網(wǎng)絡(luò)參數(shù),求梯度過程通常是一件非常復(fù)雜而容易出錯的事情。
而深度學(xué)習(xí)框架可以幫助我們自動地完成這種求梯度運算。
Pytorch一般通過反向傳播 backward方法 實現(xiàn)這種求梯度計算。該方法求得的梯度將存在對應(yīng)自變量張量的grad屬性下。
除此之外,也能夠調(diào)用torch.autograd.grad函數(shù)來實現(xiàn)求梯度計算。
這就是Pytorch的自動微分機制。
二、利用backward方法求導(dǎo)數(shù)
backward方法通常在一個標量張量上調(diào)用,該方法求得的梯度將存在對應(yīng)自變量張量的grad屬性下。如果調(diào)用的張量非標量,則要傳入一個和它同形狀的gradient參數(shù)張量。相當于用該gradient參數(shù)張量與調(diào)用張量作向量點乘,得到的標量結(jié)果再反向傳播。
1, 標量的反向傳播
import numpy as np
import torch
# f(x) = a*x**2 + b*x + c的導(dǎo)數(shù)
x = torch.tensor(0.0,requires_grad = True) # x需要被求導(dǎo)
a = torch.tensor(1.0)
b = torch.tensor(-2.0)
c = torch.tensor(1.0)
y = a*torch.pow(x,2) + b*x + c
y.backward()
dy_dx = x.grad
print(dy_dx)
輸出:
tensor(-2.)
2, 非標量的反向傳播
import numpy as np
import torch
# f(x) = a*x**2 + b*x + c
x = torch.tensor([[0.0,0.0],[1.0,2.0]],requires_grad = True) # x需要被求導(dǎo)
a = torch.tensor(1.0)
b = torch.tensor(-2.0)
c = torch.tensor(1.0)
y = a*torch.pow(x,2) + b*x + c
gradient = torch.tensor([[1.0,1.0],[1.0,1.0]])
print("x:\n",x)
print("y:\n",y)
y.backward(gradient = gradient)
x_grad = x.grad
print("x_grad:\n",x_grad)
輸出:
x:
tensor([[0., 0.],
[1., 2.]], requires_grad=True)
y:
tensor([[1., 1.],
[0., 1.]], grad_fn=AddBackward0>)
x_grad:
tensor([[-2., -2.],
[ 0., 2.]])
3, 非標量的反向傳播可以用標量的反向傳播實現(xiàn)
import numpy as np
import torch
# f(x) = a*x**2 + b*x + c
x = torch.tensor([[0.0,0.0],[1.0,2.0]],requires_grad = True) # x需要被求導(dǎo)
a = torch.tensor(1.0)
b = torch.tensor(-2.0)
c = torch.tensor(1.0)
y = a*torch.pow(x,2) + b*x + c
gradient = torch.tensor([[1.0,1.0],[1.0,1.0]])
z = torch.sum(y*gradient)
print("x:",x)
print("y:",y)
z.backward()
x_grad = x.grad
print("x_grad:\n",x_grad)
輸出:
x: tensor([[0., 0.],
[1., 2.]], requires_grad=True)
y: tensor([[1., 1.],
[0., 1.]], grad_fn=AddBackward0>)
x_grad:
tensor([[-2., -2.],
[ 0., 2.]])
三、利用autograd.grad方法求導(dǎo)數(shù)
import numpy as np
import torch
# f(x) = a*x**2 + b*x + c的導(dǎo)數(shù)
x = torch.tensor(0.0,requires_grad = True) # x需要被求導(dǎo)
a = torch.tensor(1.0)
b = torch.tensor(-2.0)
c = torch.tensor(1.0)
y = a*torch.pow(x,2) + b*x + c
# create_graph 設(shè)置為 True 將允許創(chuàng)建更高階的導(dǎo)數(shù)
dy_dx = torch.autograd.grad(y,x,create_graph=True)[0]
print(dy_dx.data)
# 求二階導(dǎo)數(shù)
dy2_dx2 = torch.autograd.grad(dy_dx,x)[0]
print(dy2_dx2.data)
輸出:
tensor(-2.)
tensor(2.)
import numpy as np
import torch
x1 = torch.tensor(1.0,requires_grad = True) # x需要被求導(dǎo)
x2 = torch.tensor(2.0,requires_grad = True)
y1 = x1*x2
y2 = x1+x2
# 允許同時對多個自變量求導(dǎo)數(shù)
(dy1_dx1,dy1_dx2) = torch.autograd.grad(outputs=y1,
inputs = [x1,x2],retain_graph = True)
print(dy1_dx1,dy1_dx2)
# 如果有多個因變量,相當于把多個因變量的梯度結(jié)果求和
(dy12_dx1,dy12_dx2) = torch.autograd.grad(outputs=[y1,y2],
inputs = [x1,x2])
print(dy12_dx1,dy12_dx2)
輸出:
tensor(2.) tensor(1.)
tensor(3.) tensor(2.)
四、利用自動微分和優(yōu)化器求最小值
import numpy as np
import torch
# f(x) = a*x**2 + b*x + c的最小值
x = torch.tensor(0.0,requires_grad = True) # x需要被求導(dǎo)
a = torch.tensor(1.0)
b = torch.tensor(-2.0)
c = torch.tensor(1.0)
optimizer = torch.optim.SGD(params=[x],lr = 0.01)
def f(x):
result = a*torch.pow(x,2) + b*x + c
return(result)
for i in range(500):
optimizer.zero_grad()
y = f(x)
y.backward()
optimizer.step()
print("y=",f(x).data,";","x=",x.data)
輸出:
y= tensor(0.) ; x= tensor(1.0000)
以上為個人經(jīng)驗,希望能給大家一個參考,也希望大家多多支持腳本之家。如有錯誤或未考慮完全的地方,望不吝賜教。
您可能感興趣的文章:- pytorch-autograde-計算圖的特點說明
- 淺談Pytorch中autograd的若干(踩坑)總結(jié)
- PyTorch一小時掌握之a(chǎn)utograd機制篇