最近遇到這個函數(shù),但查的中文博客里的解釋貌似不是很到位,這里翻譯一下stackoverflow上的回答并加上自己的理解。
在pytorch中,只有很少幾個操作是不改變tensor的內(nèi)容本身,而只是重新定義下標與元素的對應(yīng)關(guān)系的。換句話說,這種操作不進行數(shù)據(jù)拷貝和數(shù)據(jù)的改變,變的是元數(shù)據(jù)。
這些操作是:
narrow(),view(),expand()和transpose()
舉個栗子,在使用transpose()進行轉(zhuǎn)置操作時,pytorch并不會創(chuàng)建新的、轉(zhuǎn)置后的tensor,而是修改了tensor中的一些屬性(也就是元數(shù)據(jù)),使得此時的offset和stride是與轉(zhuǎn)置tensor相對應(yīng)的。
轉(zhuǎn)置的tensor和原tensor的內(nèi)存是共享的!
為了證明這一點,我們來看下面的代碼:
x = torch.randn(3, 2)
y = x.transpose(x, 0, 1)
x[0, 0] = 233
print(y[0, 0])
# print 233
可以看到,改變了y的元素的值的同時,x的元素的值也發(fā)生了變化。
也就是說,經(jīng)過上述操作后得到的tensor,它內(nèi)部數(shù)據(jù)的布局方式和從頭開始創(chuàng)建一個這樣的常規(guī)的tensor的布局方式是不一樣的!于是…這就有contiguous()的用武之地了。
在上面的例子中,x是contiguous的,但y不是(因為內(nèi)部數(shù)據(jù)不是通常的布局方式)。
注意不要被contiguous的字面意思“連續(xù)的”誤解,tensor中數(shù)據(jù)還是在內(nèi)存中一塊區(qū)域里,只是布局的問題!
當調(diào)用contiguous()時,會強制拷貝一份tensor,讓它的布局和從頭創(chuàng)建的一毛一樣。
一般來說這一點不用太擔心,如果你沒在需要調(diào)用contiguous()的地方調(diào)用contiguous(),運行時會提示你:
RuntimeError: input is not contiguous
只要看到這個錯誤提示,加上contiguous()就好啦~
補充:pytorch之expand,gather,squeeze,sum,contiguous,softmax,max,argmax
gather
torch.gather(input,dim,index,out=None)。對指定維進行索引。比如4*3的張量,對dim=1進行索引,那么index的取值范圍就是0~2.
input是一個張量,index是索引張量。input和index的size要么全部維度都相同,要么指定的dim那一維度值不同。輸出為和index大小相同的張量。
import torch
a=torch.tensor([[.1,.2,.3],
[1.1,1.2,1.3],
[2.1,2.2,2.3],
[3.1,3.2,3.3]])
b=torch.LongTensor([[1,2,1],
[2,2,2],
[2,2,2],
[1,1,0]])
b=b.view(4,3)
print(a.gather(1,b))
print(a.gather(0,b))
c=torch.LongTensor([1,2,0,1])
c=c.view(4,1)
print(a.gather(1,c))
輸出:
tensor([[ 0.2000, 0.3000, 0.2000],
[ 1.3000, 1.3000, 1.3000],
[ 2.3000, 2.3000, 2.3000],
[ 3.2000, 3.2000, 3.1000]])
tensor([[ 1.1000, 2.2000, 1.3000],
[ 2.1000, 2.2000, 2.3000],
[ 2.1000, 2.2000, 2.3000],
[ 1.1000, 1.2000, 0.3000]])
tensor([[ 0.2000],
[ 1.3000],
[ 2.1000],
[ 3.2000]])
squeeze
將維度為1的壓縮掉。如size為(3,1,1,2),壓縮之后為(3,2)
import torch
a=torch.randn(2,1,1,3)
print(a)
print(a.squeeze())
輸出:
tensor([[[[-0.2320, 0.9513, 1.1613]]],
[[[ 0.0901, 0.9613, -0.9344]]]])
tensor([[-0.2320, 0.9513, 1.1613],
[ 0.0901, 0.9613, -0.9344]])
expand
擴展某個size為1的維度。如(2,2,1)擴展為(2,2,3)
import torch
x=torch.randn(2,2,1)
print(x)
y=x.expand(2,2,3)
print(y)
輸出:
tensor([[[ 0.0608],
[ 2.2106]],
[[-1.9287],
[ 0.8748]]])
tensor([[[ 0.0608, 0.0608, 0.0608],
[ 2.2106, 2.2106, 2.2106]],
[[-1.9287, -1.9287, -1.9287],
[ 0.8748, 0.8748, 0.8748]]])
sum
size為(m,n,d)的張量,dim=1時,輸出為size為(m,d)的張量
import torch
a=torch.tensor([[[1,2,3],[4,8,12]],[[1,2,3],[4,8,12]]])
print(a.sum())
print(a.sum(dim=1))
輸出:
tensor(60)
tensor([[ 5, 10, 15],
[ 5, 10, 15]])
contiguous
返回一個內(nèi)存為連續(xù)的張量,如本身就是連續(xù)的,返回它自己。一般用在view()函數(shù)之前,因為view()要求調(diào)用張量是連續(xù)的。
可以通過is_contiguous查看張量內(nèi)存是否連續(xù)。
import torch
a=torch.tensor([[[1,2,3],[4,8,12]],[[1,2,3],[4,8,12]]])
print(a.is_contiguous)
print(a.contiguous().view(4,3))
輸出:
built-in method is_contiguous of Tensor object at 0x7f4b5e35afa0>
tensor([[ 1, 2, 3],
[ 4, 8, 12],
[ 1, 2, 3],
[ 4, 8, 12]])
softmax
假設(shè)數(shù)組V有C個元素。對其進行softmax等價于將V的每個元素的指數(shù)除以所有元素的指數(shù)之和。這會使值落在區(qū)間(0,1)上,并且和為1。
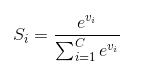
import torch
import torch.nn.functional as F
a=torch.tensor([[1.,1],[2,1],[3,1],[1,2],[1,3]])
b=F.softmax(a,dim=1)
print(b)
輸出:
tensor([[ 0.5000, 0.5000],
[ 0.7311, 0.2689],
[ 0.8808, 0.1192],
[ 0.2689, 0.7311],
[ 0.1192, 0.8808]])
max
返回最大值,或指定維度的最大值以及index
import torch
a=torch.tensor([[.1,.2,.3],
[1.1,1.2,1.3],
[2.1,2.2,2.3],
[3.1,3.2,3.3]])
print(a.max(dim=1))
print(a.max())
輸出:
(tensor([ 0.3000, 1.3000, 2.3000, 3.3000]), tensor([ 2, 2, 2, 2]))
tensor(3.3000)
argmax
返回最大值的index
import torch
a=torch.tensor([[.1,.2,.3],
[1.1,1.2,1.3],
[2.1,2.2,2.3],
[3.1,3.2,3.3]])
print(a.argmax(dim=1))
print(a.argmax())
輸出:
tensor([ 2, 2, 2, 2])
tensor(11)
以上為個人經(jīng)驗,希望能給大家一個參考,也希望大家多多支持腳本之家。如有錯誤或未考慮完全的地方,望不吝賜教。
您可能感興趣的文章:- Pytorch之contiguous的用法
- 基于pytorch中的Sequential用法說明
- Pytorch Tensor基本數(shù)學運算詳解
- 詳解PyTorch中Tensor的高階操作