前面給大家分享了pandas.merge用法詳解,這節(jié)分享pandas數(shù)據(jù)合并處理的姊妹篇,pandas.concat用法詳解,參考利用Python進(jìn)行數(shù)據(jù)分析與pandas官網(wǎng)進(jìn)行整理。
pandas.merge參數(shù)列表如下圖,其中只有objs是必須得參數(shù),另外常用參數(shù)包括objs、axis、join、keys、ignore_index。
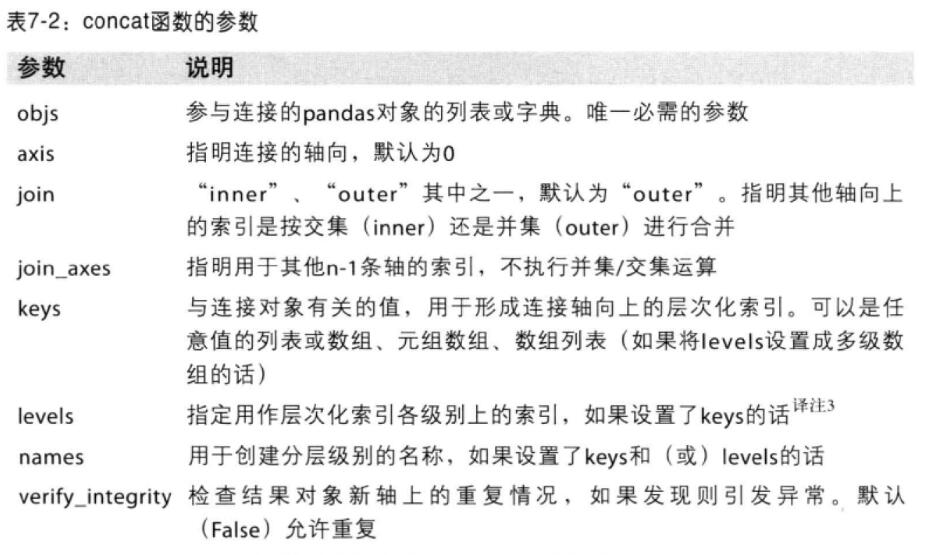
1.pd.concat([df1,df2,df3])
, 默認(rèn)axis=0,在0軸上合并。
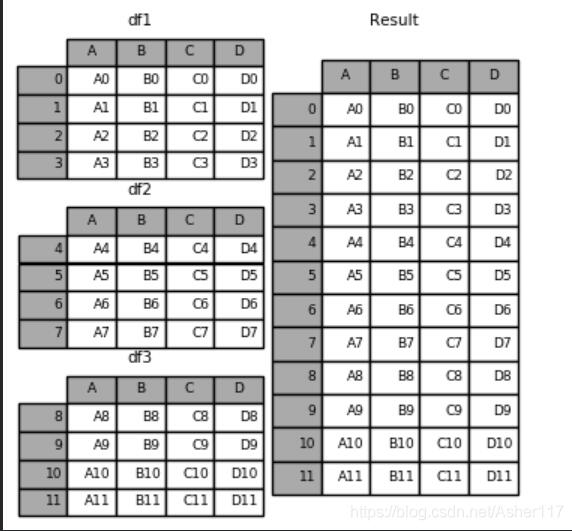
2.pd.concat([df1,df4],axis=1)
–在1軸上合并
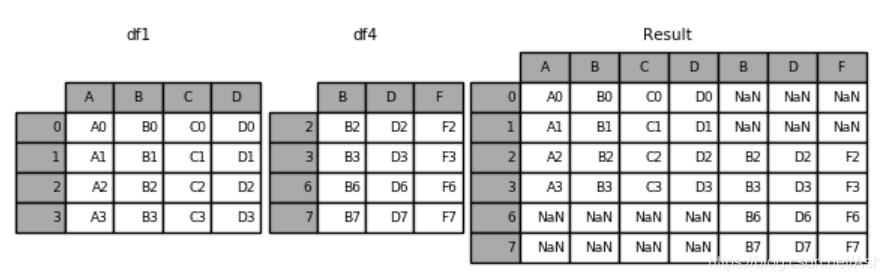
3.pd.concat([df1,df2,df3],keys=[‘x', ‘y', ‘z'])
–合并時(shí)便于區(qū)分建立層次化索引。
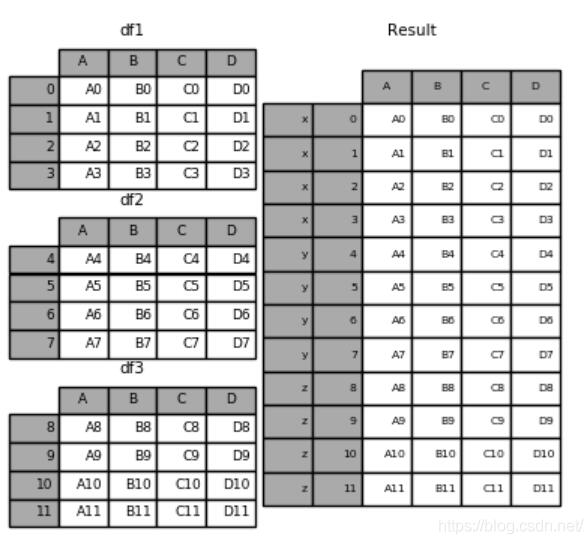
4.pd.concat([df1, df4], axis=1, join=‘inner')
–采用內(nèi)連接合并,join默認(rèn)為outer外連接。
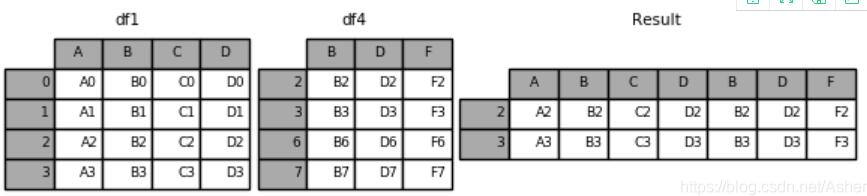
5.pd.concat([df1, df4], ignore_index=True)
–當(dāng)原來DataFrame的索引沒有意義的時(shí)候,concat之后可以不需要原來的索引。
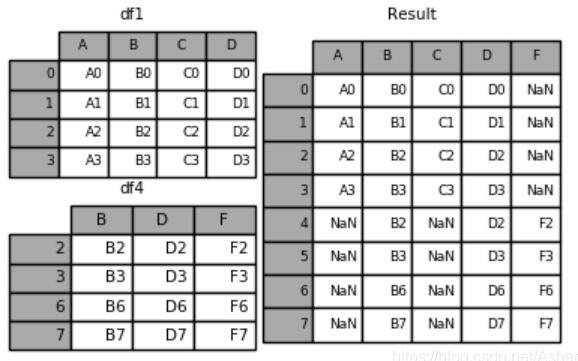
姊妹篇:pandas.merge用法詳解!??!
補(bǔ)充:python3:pandas(合并concat和merge)
pandas處理多組數(shù)據(jù)的時(shí)候往往會(huì)要用到數(shù)據(jù)的合并處理,其中有三種方式,concat、append和merge。
1、concat
用concat是一種基本的合并方式。而且concat中有很多參數(shù)可以調(diào)整,合并成你想要的數(shù)據(jù)形式。axis來指明合并方向。axis=0是預(yù)設(shè)值,因此未設(shè)定任何參數(shù)時(shí),函數(shù)默認(rèn)axis=0。(0表示上下合并,1表示左右合并)
import pandas as pd
import numpy as np
#定義資料集
df1 = pd.DataFrame(np.ones((3,4))*0, columns=['a','b','c','d'])
df2 = pd.DataFrame(np.ones((3,4))*1, columns=['a','b','c','d'])
df3 = pd.DataFrame(np.ones((3,4))*2, columns=['a','b','c','d'])
#concat縱向合并
res = pd.concat([df1, df2, df3], axis=0)
#打印結(jié)果
print(res)
'''
a b c d
0 0.0 0.0 0.0 0.0
1 0.0 0.0 0.0 0.0
2 0.0 0.0 0.0 0.0
0 1.0 1.0 1.0 1.0
1 1.0 1.0 1.0 1.0
2 1.0 1.0 1.0 1.0
0 2.0 2.0 2.0 2.0
1 2.0 2.0 2.0 2.0
2 2.0 2.0 2.0 2.0
'''
上述index為0,1,2,0,1,2形式。為什么會(huì)出現(xiàn)這樣的情況,其實(shí)是仍然按照合并前的index組合起來的。若希望遞增,請(qǐng)看下面示例:
ignore_index (重置 index)
重置后的index為0,1,……8
res = pd.concat([df1, df2, df3], axis=0, ignore_index=True)# 將ignore_index設(shè)置為True
print(res) #打印結(jié)果
'''
a b c d
0 0.0 0.0 0.0 0.0
1 0.0 0.0 0.0 0.0
2 0.0 0.0 0.0 0.0
3 1.0 1.0 1.0 1.0
4 1.0 1.0 1.0 1.0
5 1.0 1.0 1.0 1.0
6 2.0 2.0 2.0 2.0
7 2.0 2.0 2.0 2.0
8 2.0 2.0 2.0 2.0
'''
join (合并方式)
join='outer'為預(yù)設(shè)值,因此未設(shè)定任何參數(shù)時(shí),函數(shù)默認(rèn)join='outer'。此方式是依照column來做縱向合并,有相同的column上下合并在一起,其他獨(dú)自的column個(gè)自成列,原本沒有值的位置皆以NaN填充。
import pandas as pd
import numpy as np
#定義資料集
df1 = pd.DataFrame(np.ones((3,4))*0, columns=['a','b','c','d'], index=[1,2,3])
df2 = pd.DataFrame(np.ones((3,4))*1, columns=['b','c','d','e'], index=[2,3,4])
res = pd.concat([df1, df2], axis=0, join='outer') #縱向"外"合并df1與df2
print(res)
'''
a b c d e
1 0.0 0.0 0.0 0.0 NaN
2 0.0 0.0 0.0 0.0 NaN
3 0.0 0.0 0.0 0.0 NaN
2 NaN 1.0 1.0 1.0 1.0
3 NaN 1.0 1.0 1.0 1.0
4 NaN 1.0 1.0 1.0 1.0
'''
res = pd.concat([df1, df2], axis=0, join='inner') #縱向"內(nèi)"合并df1與df2
#打印結(jié)果
print(res)
'''
b c d
1 0.0 0.0 0.0
2 0.0 0.0 0.0
3 0.0 0.0 0.0
2 1.0 1.0 1.0
3 1.0 1.0 1.0
4 1.0 1.0 1.0
'''
join_axes (依照 axes 合并)
import pandas as pd
import numpy as np
#定義資料集
df1 = pd.DataFrame(np.ones((3,4))*0, columns=['a','b','c','d'], index=[1,2,3])
df2 = pd.DataFrame(np.ones((3,4))*1, columns=['b','c','d','e'], index=[2,3,4])
#依照`df1.index`進(jìn)行橫向合并
res = pd.concat([df1, df2], axis=1, join_axes=[df1.index])
#打印結(jié)果
print(res)
# a b c d b c d e
# 1 0.0 0.0 0.0 0.0 NaN NaN NaN NaN
# 2 0.0 0.0 0.0 0.0 1.0 1.0 1.0 1.0
# 3 0.0 0.0 0.0 0.0 1.0 1.0 1.0 1.0
上述腳本中,join_axes=[df1.index]表明按照df1的index來合并,可以看到結(jié)果中去掉了df2中出現(xiàn)但df1中沒有的index=4這一行。
2、append (添加數(shù)據(jù))
append只有縱向合并,沒有橫向合并。
import pandas as pd
import numpy as np
#定義資料集
df1 = pd.DataFrame(np.ones((3,4))*0, columns=['a','b','c','d'])
df2 = pd.DataFrame(np.ones((3,4))*1, columns=['a','b','c','d'])
df3 = pd.DataFrame(np.ones((3,4))*1, columns=['a','b','c','d'])
s1 = pd.Series([1,2,3,4], index=['a','b','c','d'])
#將df2合并到df1的下面,以及重置index,并打印出結(jié)果
res = df1.append(df2, ignore_index=True)
print(res)
# a b c d
# 0 0.0 0.0 0.0 0.0
# 1 0.0 0.0 0.0 0.0
# 2 0.0 0.0 0.0 0.0
# 3 1.0 1.0 1.0 1.0
# 4 1.0 1.0 1.0 1.0
# 5 1.0 1.0 1.0 1.0
#合并多個(gè)df,將df2與df3合并至df1的下面,以及重置index,并打印出結(jié)果
res = df1.append([df2, df3], ignore_index=True)
print(res)
# a b c d
# 0 0.0 0.0 0.0 0.0
# 1 0.0 0.0 0.0 0.0
# 2 0.0 0.0 0.0 0.0
# 3 1.0 1.0 1.0 1.0
# 4 1.0 1.0 1.0 1.0
# 5 1.0 1.0 1.0 1.0
# 6 1.0 1.0 1.0 1.0
# 7 1.0 1.0 1.0 1.0
# 8 1.0 1.0 1.0 1.0
#合并series,將s1合并至df1,以及重置index,并打印出結(jié)果
res = df1.append(s1, ignore_index=True)
print(res)
# a b c d
# 0 0.0 0.0 0.0 0.0
# 1 0.0 0.0 0.0 0.0
# 2 0.0 0.0 0.0 0.0
# 3 1.0 2.0 3.0 4.0
3、merge
根據(jù)兩組數(shù)據(jù)中的關(guān)鍵字key來合并(key在兩組數(shù)據(jù)中是完全一致的)。
3.1依據(jù)一組key合并
import pandas as pd
#定義資料集并打印出
left = pd.DataFrame({'key': ['K0', 'K1', 'K2', 'K3'],
'A': ['A0', 'A1', 'A2', 'A3'],
'B': ['B0', 'B1', 'B2', 'B3']})
right = pd.DataFrame({'key': ['K0', 'K1', 'K2', 'K3'],
'C': ['C0', 'C1', 'C2', 'C3'],
'D': ['D0', 'D1', 'D2', 'D3']})
print(left)
# A B key
# 0 A0 B0 K0
# 1 A1 B1 K1
# 2 A2 B2 K2
# 3 A3 B3 K3
print(right)
# C D key
# 0 C0 D0 K0
# 1 C1 D1 K1
# 2 C2 D2 K2
# 3 C3 D3 K3
#依據(jù)key column合并,并打印出
res = pd.merge(left, right, on='key')
print(res)
A B key C D
# 0 A0 B0 K0 C0 D0
# 1 A1 B1 K1 C1 D1
# 2 A2 B2 K2 C2 D2
# 3 A3 B3 K3 C3 D3
3.2 根據(jù)兩組key合并
合并時(shí)有4種方法how = ['left', 'right', 'outer', 'inner'],預(yù)設(shè)值how='inner'。
inner:按照關(guān)鍵字組合之后,去掉組合中有合并項(xiàng)為NaN的行。
outer :保留所有組合
left:僅保留左邊合并項(xiàng)為NaN的行
right:僅保留右邊合并項(xiàng)為NaN的行
import pandas as pd
import numpy as np
#定義資料集并打印出
left = pd.DataFrame({'key1': ['K0', 'K0', 'K1', 'K2'],
'key2': ['K0', 'K1', 'K0', 'K1'],
'A': ['A0', 'A1', 'A2', 'A3'],
'B': ['B0', 'B1', 'B2', 'B3']})
right = pd.DataFrame({'key1': ['K0', 'K1', 'K1', 'K2'],
'key2': ['K0', 'K0', 'K0', 'K0'],
'C': ['C0', 'C1', 'C2', 'C3'],
'D': ['D0', 'D1', 'D2', 'D3']})
print(left)
'''
key1 key2 A B
0 K0 K0 A0 B0
1 K0 K1 A1 B1
2 K1 K0 A2 B2
3 K2 K1 A3 B3
'''
print(right)
'''
key1 key2 C D
0 K0 K0 C0 D0
1 K1 K0 C1 D1
2 K1 K0 C2 D2
3 K2 K0 C3 D3
'''
#依據(jù)key1與key2 columns進(jìn)行合并,并打印出四種結(jié)果['left', 'right', 'outer', 'inner']
res = pd.merge(left, right, on=['key1', 'key2'], how='inner')
print(res)
'''
key1 key2 A B C D
0 K0 K0 A0 B0 C0 D0
1 K1 K0 A2 B2 C1 D1
2 K1 K0 A2 B2 C2 D2
'''
res = pd.merge(left, right, on=['key1', 'key2'], how='outer')
print(res)
'''
key1 key2 A B C D
0 K0 K0 A0 B0 C0 D0
1 K0 K1 A1 B1 NaN NaN
2 K1 K0 A2 B2 C1 D1
3 K1 K0 A2 B2 C2 D2
4 K2 K1 A3 B3 NaN NaN
5 K2 K0 NaN NaN C3 D3
'''
res = pd.merge(left, right, on=['key1', 'key2'], how='left')
print(res)
'''
key1 key2 A B C D
0 K0 K0 A0 B0 C0 D0
1 K0 K1 A1 B1 NaN NaN
2 K1 K0 A2 B2 C1 D1
3 K1 K0 A2 B2 C2 D2
4 K2 K1 A3 B3 NaN NaN
'''
res = pd.merge(left, right, on=['key1', 'key2'], how='right')
print(res)
'''
key1 key2 A B C D
0 K0 K0 A0 B0 C0 D0
1 K1 K0 A2 B2 C1 D1
2 K1 K0 A2 B2 C2 D2
3 K2 K0 NaN NaN C3 D3
'''
3.3 Indicator
indicator=True會(huì)將合并的記錄放在新的一列。
import pandas as pd
#定義資料集并打印出
df1 = pd.DataFrame({'col1':[0,1], 'col_left':['a','b']})
df2 = pd.DataFrame({'col1':[1,2,2],'col_right':[2,2,2]})
print(df1)
# col1 col_left
# 0 0 a
# 1 1 b
print(df2)
# col1 col_right
# 0 1 2
# 1 2 2
# 2 2 2
# 依據(jù)col1進(jìn)行合并,并啟用indicator=True,最后打印出
res = pd.merge(df1, df2, on='col1', how='outer', indicator=True)
print(res)
# col1 col_left col_right _merge
# 0 0.0 a NaN left_only
# 1 1.0 b 2.0 both
# 2 2.0 NaN 2.0 right_only
# 3 2.0 NaN 2.0 right_only
# 自定indicator column的名稱,并打印出
res = pd.merge(df1, df2, on='col1', how='outer', indicator='indicator_column')
print(res)
# col1 col_left col_right indicator_column
# 0 0.0 a NaN left_only
# 1 1.0 b 2.0 both
# 2 2.0 NaN 2.0 right_only
# 3 2.0 NaN 2.0 right_only
3.4 依據(jù)index合并
import pandas as pd
#定義資料集并打印出
left = pd.DataFrame({'A': ['A0', 'A1', 'A2'],
'B': ['B0', 'B1', 'B2']},
index=['K0', 'K1', 'K2'])
right = pd.DataFrame({'C': ['C0', 'C2', 'C3'],
'D': ['D0', 'D2', 'D3']},
index=['K0', 'K2', 'K3'])
print(left)
# A B
# K0 A0 B0
# K1 A1 B1
# K2 A2 B2
print(right)
# C D
# K0 C0 D0
# K2 C2 D2
# K3 C3 D3
#依據(jù)左右資料集的index進(jìn)行合并,how='outer',并打印出
res = pd.merge(left, right, left_index=True, right_index=True, how='outer')
print(res)
# A B C D
# K0 A0 B0 C0 D0
# K1 A1 B1 NaN NaN
# K2 A2 B2 C2 D2
# K3 NaN NaN C3 D3
#依據(jù)左右資料集的index進(jìn)行合并,how='inner',并打印出
res = pd.merge(left, right, left_index=True, right_index=True, how='inner')
print(res)
# A B C D
# K0 A0 B0 C0 D0
# K2 A2 B2 C2 D2
3.5 解決overlapping的問題
下面腳本中,boys和girls均有屬性age,但是兩者值不同,因此需要在合并時(shí)加上后綴suffixes,以示區(qū)分。
import pandas as pd
#定義資料集
boys = pd.DataFrame({'k': ['K0', 'K1', 'K2'], 'age': [1, 2, 3]})
girls = pd.DataFrame({'k': ['K0', 'K0', 'K3'], 'age': [4, 5, 6]})
#使用suffixes解決overlapping的問題
res = pd.merge(boys, girls, on='k', suffixes=['_boy', '_girl'], how='inner')
print(res)
# age_boy k age_girl
# 0 1 K0 4
# 1 1 K0 5
以上是pandas中有關(guān)于合并的一些操作。當(dāng)然,如果練習(xí)的多了,幾個(gè)方法也是大同小異。希望大家多多支持腳本之家。如有錯(cuò)誤或未考慮完全的地方,望不吝賜教。
您可能感興趣的文章:- 利用python Pandas實(shí)現(xiàn)批量拆分Excel與合并Excel
- 解決python3安裝pandas出錯(cuò)的問題
- Python機(jī)器學(xué)習(xí)三大件之二pandas
- Python Pandas知識(shí)點(diǎn)之缺失值處理詳解
- Python基礎(chǔ)之pandas數(shù)據(jù)合并
- python基于Pandas讀寫MySQL數(shù)據(jù)庫(kù)
- python pandas合并Sheet,處理列亂序和出現(xiàn)Unnamed列的解決
- python 使用pandas同時(shí)對(duì)多列進(jìn)行賦值
- python pandas模糊匹配 讀取Excel后 獲取指定指標(biāo)的操作
- Python數(shù)據(jù)分析之pandas讀取數(shù)據(jù)