目錄
- 寫在之前
- 一、代碼框架
- 二、準(zhǔn)備工作
- 三、框架的開始
- 四、訓(xùn)練模型構(gòu)建
- 五、手寫數(shù)字的識別
- 六、源碼
- 七、思考
寫在之前
首先是寫在之前的一些建議:
首先是關(guān)于這本書,我真的認(rèn)為他是將神經(jīng)網(wǎng)絡(luò)里非常棒的一本書,但你也需要注意,如果你真的想自己動手去實現(xiàn),那么你一定需要有一定的python基礎(chǔ),并且還需要有一些python數(shù)據(jù)科學(xué)處理能力
然后希望大家在看這邊博客的時候?qū)τ谏窠?jīng)網(wǎng)絡(luò)已經(jīng)有一些了解了,知道什么是輸入層,什么是輸出層,并且明白他們的一些理論,在這篇博客中我們僅僅是展開一下代碼;
然后介紹一下本篇博客的環(huán)境等:
語言:Python3.8.5
環(huán)境:jupyter
庫文件: numpy | matplotlib | scipy
一、代碼框架
我們即將設(shè)計一個神經(jīng)網(wǎng)絡(luò)對象,它可以幫我們?nèi)プ鰯?shù)據(jù)的訓(xùn)練,以及數(shù)據(jù)的預(yù)測,所以我們將具有以下的三個方法:
首先我們需要初始化這個函數(shù),我們希望這個神經(jīng)網(wǎng)絡(luò)僅有三層,因為再多也不過是在隱藏層去做文章,所以先做一個簡單的。那么我們需要知道我們輸入層、隱藏層和輸出層的節(jié)點個數(shù);訓(xùn)練函數(shù),我們需要去做訓(xùn)練,得到我們需要的權(quán)重。通過我們已有的權(quán)重,將給定的輸入去做輸出。
二、準(zhǔn)備工作
現(xiàn)在我們需要準(zhǔn)備一下:
1.將我們需要的庫導(dǎo)入
import numpy as np
import scipy.special as spe
import matplotlib.pyplot as plt
2.構(gòu)建一個類
class neuralnetwork:
# 我們需要去初始化一個神經(jīng)網(wǎng)絡(luò)
def __init__(self, inputnodes, hiddennodes, outputnodes, learningrate):
pass
def train(self, inputs_list, targets_list):
pass
def query(self, inputs_list):
pass
3.我們的主函數(shù)
input_nodes = 784 # 輸入層的節(jié)點數(shù)
hidden_nodes = 88 # 隱藏層的節(jié)點數(shù)
output_nodes = 10 # 輸出層的節(jié)點數(shù)
learn_rate = 0.05 # 學(xué)習(xí)率
n = neuralnetwork(input_nodes, hidden_nodes, output_nodes, learn_rate)
4.導(dǎo)入文件
data_file = open("E:\sklearn_data\神經(jīng)網(wǎng)絡(luò)數(shù)字識別\mnist_train.csv", 'r')
data_list = data_file.readlines()
data_file.close()
file2 = open("E:\sklearn_data\神經(jīng)網(wǎng)絡(luò)數(shù)字識別\mnist_test.csv")
answer_data = file2.readlines()
file2.close()
這里需要介紹以下這個數(shù)據(jù)集,訓(xùn)練集在這里,測試集在這里
三、框架的開始
def __init__(self, inputnodes, hiddennodes, outputnodes, learningrate):
self.inodes = inputnodes # 輸入層節(jié)點設(shè)定
self.hnodes = hiddennodes # 影藏層節(jié)點設(shè)定
self.onodes = outputnodes # 輸出層節(jié)點設(shè)定
self.lr = learningrate # 學(xué)習(xí)率設(shè)定,這里可以改進(jìn)的
self.wih = (np.random.normal(0.0, pow(self.hnodes, -0.5),(self.hnodes, self.inodes))) # 這里是輸入層與隱藏層之間的連接
self.who = (np.random.normal(0.0, pow(self.onodes, -0.5),(self.onodes, self.hnodes))) # 這里是隱藏層與輸出層之間的連接
self.activation_function = lambda x: spe.expit(x) # 返回sigmoid函數(shù)
Δw j,k =α∗E k ∗ sigmoid (O k )∗(1−sigmoid(O k ))⋅O j ⊤
def query(self, inputs_list):
inputs = np.array(inputs_list, ndmin=2).T # 輸入進(jìn)來的二維圖像數(shù)據(jù)
hidden_inputs = np.dot(self.wih, inputs) # 隱藏層計算,說白了就是線性代數(shù)中的矩陣的點積
hidden_outputs = self.activation_function(hidden_inputs) # 將隱藏層的輸出是經(jīng)過sigmoid函數(shù)處理
final_inputs = np.dot(self.who, hidden_outputs) # 原理同hidden_inputs
final_outputs = self.activation_function(final_inputs) # 原理同hidden_outputs
return final_outputs # 最終的輸出結(jié)果就是我們預(yù)測的數(shù)據(jù)
這里我們對預(yù)測這一部分做一個簡單的解釋:我們之前的定義輸出的節(jié)點是10個,對應(yīng)的是十個數(shù)字。
而為什么會通過神經(jīng)網(wǎng)絡(luò)能達(dá)到這個亞子,我推薦這本書深度學(xué)習(xí)的數(shù)學(xué) 這本書的理論講解非常不錯?。。?/p>
四、訓(xùn)練模型構(gòu)建
之前的部分相對而言還是比較簡單的,那么接下來就是如何去構(gòu)建訓(xùn)練模型了。
def train(self, inputs_list, targets_list):
# 前期和識別過程是一樣的,說白了我們與要先看看現(xiàn)在的預(yù)測結(jié)果如何,只有根據(jù)這次的預(yù)期結(jié)果才能去修改之前的權(quán)重
inputs = np.array(inputs_list, ndmin=2).T
hidden_inputs = np.dot(self.wih, inputs)
hidden_outputs = self.activation_function(hidden_inputs)
final_inputs = np.dot(self.who, hidden_outputs)
final_outputs = self.activation_function(final_inputs)
# 接下來將標(biāo)簽?zāi)眠t來
targets = np.array(targets_list, ndmin=2).T
# 得到我們的數(shù)據(jù)預(yù)測的誤差,這個誤差將是向前反饋的基礎(chǔ)
output_errors = targets - final_outputs
# 這部分是根據(jù)公式得到的反向傳播參數(shù)
hidden_errors = np.dot(self.who.T, output_errors)
# 根據(jù)我們的反饋參數(shù)去修改兩個權(quán)重
self.who += self.lr * np.dot((output_errors * final_outputs * ( 1.0-final_outputs)), np.transpose(hidden_outputs))
self.wih += self.lr * np.dot((hidden_errors * hidden_outputs * (1.0-hidden_outputs)), np.transpose(inputs))
如此我們的基礎(chǔ)神經(jīng)網(wǎng)絡(luò)構(gòu)建完成了。
五、手寫數(shù)字的識別
接下來神經(jīng)網(wǎng)絡(luò)是完成的,那么我們究竟該如何去將數(shù)據(jù)輸入呢?
csv文件我們并不陌生【或許陌生?】,他是逗號分割文件,顧名思義,它是通過逗號分隔的,所以我們可以打開看一下:
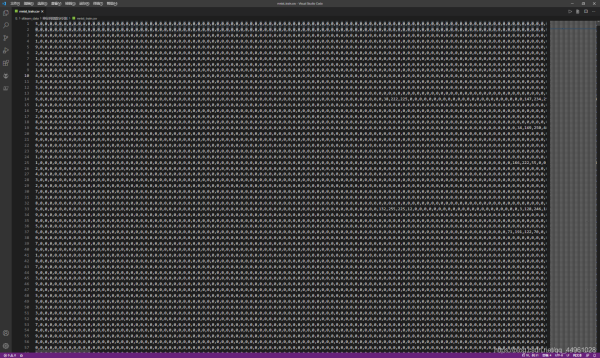
眼花繚亂?。?/p>
但是細(xì)心的我們可以發(fā)現(xiàn)他的第一個數(shù)字都是0~9,說明是我們的標(biāo)簽,那么后面的應(yīng)該就是圖像了,通過了解我們知道這個后面的數(shù)據(jù)是一個28*28的圖像。
all_value = data_list[0].split(',') # split分割成列表
image_array = np.asfarray(all_value[1:]).reshape((28,28)) # 將數(shù)據(jù)reshape成28*28的矩陣
plt.imshow(image_array, cmap='Greys', interpolation='None') # 展示一下
通過這段代碼,我們可以簡單的看一下每個數(shù)字是什么:
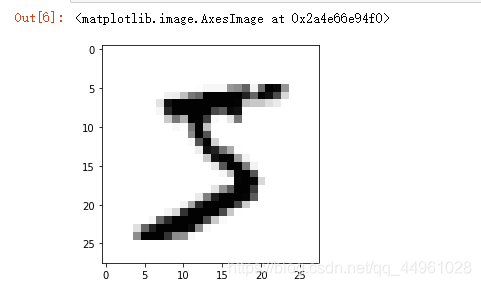
很好,知道這里就足夠了,那么我們接下來就是將這些數(shù)據(jù)傳入了!
我們在訓(xùn)練的時候,需要將他們都轉(zhuǎn)化成數(shù)字列表,方便處理
data = [] # 用來保存訓(xùn)練過程的數(shù)據(jù)
sum_count = 0 # 統(tǒng)計總識別的正確的個數(shù)
for i in range(15): # 訓(xùn)練的輪數(shù)
count = 0 # 單次訓(xùn)練識別正確的個數(shù)
for j in range(len(data_list)): # 對60000張圖片開始訓(xùn)練, 沒有劃分?jǐn)?shù)據(jù)集的過程主要是別人直接給了,我也懶得自己去做了,主要就是展示一下神經(jīng)網(wǎng)絡(luò)嘛~
target = np.zeros(10)+0.01 # 生成初始標(biāo)簽集合,用來和結(jié)果對比
line_ = data_list[j].split(',') # 對每一行的數(shù)據(jù)處理切割
imagearray = np.asfarray(line_) # 將切割完成的數(shù)據(jù)轉(zhuǎn)換成數(shù)字列表
target[int(imagearray[0])] = 1.0 # 將正確答案挑出來
n.train(imagearray[1:]/255*0.99+0.01, target) # 丟入訓(xùn)練,丟入的時候注意將數(shù)據(jù)轉(zhuǎn)換成0.01~1.0之間的結(jié)果
for line in answer_data: # 對10000組測試集測試
all_values = line.split(',')
answer = n.query((np.asfarray(all_values[1:])/255*0.99)+0.01)
if answer[int(all_values[0])] > 0.85: # 查看對應(yīng)位置是否達(dá)到自定義的閾值?
count += 1
sum_count += count
string = "訓(xùn)練進(jìn)度 %05f\n本輪準(zhǔn)確度 %05f\n總準(zhǔn)確度 %05f\n\n"%(i/120,count/len(answer_data), sum_count/(len(answer_data)*(i+1)))
data.append([i/120,count/len(answer_data), sum_count/(len(answer_data)*(i+1))]) # 將數(shù)據(jù)保存方便生成訓(xùn)練曲線
print(string)
```
接下來我們將結(jié)果圖片展示以下吧~
```python
data = np.array(data)
plt.plot(range(len(data)), data[:, 1:])
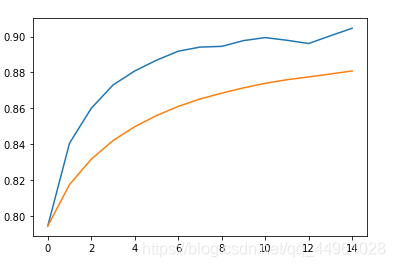
六、源碼
把源碼整理一下貼出來
import numpy as np
import scipy.special as spe
import matplotlib.pyplot as plt
class neuralnetwork:
# 我們需要去初始化一個神經(jīng)網(wǎng)絡(luò)
def __init__(self, inputnodes, hiddennodes, outputnodes, learningrate):
self.inodes = inputnodes
self.hnodes = hiddennodes
self.onodes = outputnodes
self.lr = learningrate
self.wih = (np.random.normal(0.0, pow(self.hnodes, -0.5),(self.hnodes, self.inodes)))
self.who = (np.random.normal(0.0, pow(self.onodes, -0.5),(self.onodes, self.hnodes)))
self.activation_function = lambda x: spe.expit(x) # 返回sigmoid函數(shù)
def train(self, inputs_list, targets_list):
inputs = np.array(inputs_list, ndmin=2).T
hidden_inputs = np.dot(self.wih, inputs)
hidden_outputs = self.activation_function(hidden_inputs)
final_inputs = np.dot(self.who, hidden_outputs)
final_outputs = self.activation_function(final_inputs)
targets = np.array(targets_list, ndmin=2).T
output_errors = targets - final_outputs
hidden_errors = np.dot(self.who.T, output_errors)
self.who += self.lr * np.dot((output_errors * final_outputs * ( 1.0-final_outputs)), np.transpose(hidden_outputs))
self.wih += self.lr * np.dot((hidden_errors * hidden_outputs * (1.0-hidden_outputs)), np.transpose(inputs))
def query(self, inputs_list):
inputs = np.array(inputs_list, ndmin=2).T
hidden_inputs = np.dot(self.wih, inputs)
hidden_outputs = self.activation_function(hidden_inputs)
final_inputs = np.dot(self.who, hidden_outputs)
final_outputs = self.activation_function(final_inputs)
return final_outputs
input_nodes = 784
hidden_nodes = 88
output_nodes = 10
learn_rate = 0.05
n = neuralnetwork(input_nodes, hidden_nodes, output_nodes, learn_rate)
data_file = open("E:\sklearn_data\神經(jīng)網(wǎng)絡(luò)數(shù)字識別\mnist_train.csv", 'r')
data_list = data_file.readlines()
data_file.close()
file2 = open("E:\sklearn_data\神經(jīng)網(wǎng)絡(luò)數(shù)字識別\mnist_test.csv")
answer_data = file2.readlines()
file2.close()
data = []
sum_count = 0
for i in range(15):
count = 0
for j in range(len(data_list)):
target = np.zeros(10)+0.01
line_ = data_list[j].split(',')
imagearray = np.asfarray(line_)
target[int(imagearray[0])] = 1.0
n.train(imagearray[1:]/255*0.99+0.01, target)
for line in answer_data:
all_values = line.split(',')
answer = n.query((np.asfarray(all_values[1:])/255*0.99)+0.01)
if answer[int(all_values[0])] > 0.85:
count += 1
sum_count += count
string = "訓(xùn)練進(jìn)度 %05f\n本輪準(zhǔn)確度 %05f\n總準(zhǔn)確度 %05f\n\n"%(i/120,count/len(answer_data), sum_count/(len(answer_data)*(i+1)))
data.append([i/120,count/len(answer_data), sum_count/(len(answer_data)*(i+1))])
print(string)
data = np.array(data)
plt.plot(range(len(data)), data[:, 1:])
可以說是相對簡單的一個程序,但卻是包含著神經(jīng)網(wǎng)絡(luò)最基礎(chǔ)的思想!值得好好康康~
七、思考
如何識別其他手寫字體等?
我的想法:通過圖像處理,將像素規(guī)定到相近大小【尺度放縮】
圖像大小運行速度問題
我的想法:如何快速的矩陣運算,通過C語言是否可以加速?相較于darknet這個神經(jīng)網(wǎng)絡(luò)僅有三層,運算速度并不是十分理想。當(dāng)然cuda編程對于GPU加速肯定是最好的選擇之一。
到此這篇關(guān)于python神經(jīng)網(wǎng)絡(luò)編程之手寫數(shù)字識別的文章就介紹到這了,更多相關(guān)python手寫數(shù)字識別內(nèi)容請搜索腳本之家以前的文章或繼續(xù)瀏覽下面的相關(guān)文章希望大家以后多多支持腳本之家!
您可能感興趣的文章:- numpy創(chuàng)建神經(jīng)網(wǎng)絡(luò)框架
- 教你使用Python建立任意層數(shù)的深度神經(jīng)網(wǎng)絡(luò)
- python機(jī)器學(xué)習(xí)之神經(jīng)網(wǎng)絡(luò)
- pytorch動態(tài)神經(jīng)網(wǎng)絡(luò)(擬合)實現(xiàn)
- Python如何使用神經(jīng)網(wǎng)絡(luò)進(jìn)行簡單文本分類
- pytorch之深度神經(jīng)網(wǎng)絡(luò)概念全面整理