如果你是pytorch的用戶,可能你會很熟悉pytorch生態(tài)圈中專門預(yù)處理圖像數(shù)據(jù)集的torchvision庫。
從torchtext這個(gè)名字我們也能大概猜到該庫是pytorch圈中用來預(yù)處理文本數(shù)據(jù)集的庫,但這方面的教程網(wǎng)絡(luò)上比較少,今天我就講講這個(gè)特別有用的文本分析庫。
簡介
torchtext在文本數(shù)據(jù)預(yù)處理方面特別強(qiáng)大,但我們要知道ta能做什么、不能做什么,并如何將我們的需求用torchtext實(shí)現(xiàn)。雖然torchtext是為pytorch而設(shè)計(jì)的,但是也可以與keras、tensorflow等結(jié)合使用。
官方文檔地址 https://torchtext.readthedocs.io/en/latest/index.html
# 安裝
!pip3 install torchtext
自然語言處理預(yù)處理的工作流程:
1、Train/Validation/Test數(shù)據(jù)集分割
2、文件數(shù)據(jù)導(dǎo)入(File Loading)
3、分詞(Tokenization) 文本字符串切分為詞語列表
4、構(gòu)建詞典(Vocab) 根據(jù)訓(xùn)練的預(yù)料數(shù)據(jù)集構(gòu)建詞典
5、數(shù)字映射(Numericalize/Indexify) 根據(jù)詞典,將數(shù)據(jù)從詞語映射成數(shù)字,方便機(jī)器學(xué)習(xí)
6、導(dǎo)入預(yù)訓(xùn)練好的詞向量(word vector)
7、分批(Batch) 數(shù)據(jù)集太大的話,不能一次性讓機(jī)器讀取,否則機(jī)器會內(nèi)存崩潰。解決辦法就是將大的數(shù)據(jù)集分成更小份的數(shù)據(jù)集,分批處理
8、向量映射(Embedding Lookup) 根據(jù)預(yù)處理好的詞向量數(shù)據(jù)集,將5的結(jié)果中每個(gè)詞語對應(yīng)的索引值變成 詞語向量
上面8個(gè)步驟,torchtext實(shí)現(xiàn)了2-7。第一步需要我們自己diy,好在這一步?jīng)]什么難度
"The quick fox jumped over a lazy dog."
# 分詞
["The", "quick", "fox", "jumped", "over", "a", "lazy", "dog", "."]
# 構(gòu)建詞典
{"The" -gt; 0,
"quick"-gt; 1,
"fox" -gt; 2,
...}
# 數(shù)字映射(將每個(gè)詞根據(jù)詞典映射為對應(yīng)的索引值)
[0, 1, 2, ...]
# 向量映射(按照導(dǎo)入的預(yù)訓(xùn)練好的詞向量數(shù)據(jù)集,把詞語映射成向量)
[
[0.3, 0.2, 0.5],
[0.6, 0., 0.1],
[0.8, 01., 0.4],
...
]
一、數(shù)據(jù)集分割
一般我們做機(jī)器學(xué)習(xí)會將數(shù)據(jù)分為訓(xùn)練集和測試集,而在深度學(xué)習(xí)中,需要多輪訓(xùn)練學(xué)習(xí),每次的學(xué)習(xí)過程都包括訓(xùn)練和驗(yàn)證,最后再進(jìn)行測試。所以需要將數(shù)據(jù)分成訓(xùn)練、驗(yàn)證和測試數(shù)據(jù)。
import pandas as pd
import numpy as np
def split_csv(infile, trainfile, valtestfile, seed=999, ratio=0.2):
df = pd.read_csv(infile)
df["text"] = df.text.str.replace("\n", " ")
idxs = np.arange(df.shape[0])
np.random.seed(seed)
np.random.shuffle(idxs)
val_size = int(len(idxs) * ratio)
df.iloc[idxs[:val_size], :].to_csv(valtestfile, index=False)
df.iloc[idxs[val_size:], :].to_csv(trainfile, index=False)
#先將sms_spam.csv數(shù)據(jù)分為train.csv和test.csv
split_csv(infile='data/sms_spam.csv',
trainfile='data/train.csv',
valtestfile='data/test.csv',
seed=999,
ratio=0.2)
#再將train.csv分為dataset_train.csv和dataset_valid.csv
split_csv(infile='data/train.csv',
trainfile='data/dataset_train.csv',
valtestfile='data/dataset_valid.csv',
seed=999,
ratio=0.2)
1.1 參數(shù)解讀
split_csv(infile, trainfile, valtestfile, seed, ratio)
infile:待分割的csv文件
trainfile:分割出的訓(xùn)練cs文件
valtestfile:分割出的測試或驗(yàn)證csv文件
seed:隨機(jī)種子,保證每次的隨機(jī)分割隨機(jī)性一致
ratio:測試(驗(yàn)證)集占數(shù)據(jù)的比例
經(jīng)過上面的操作,我們已經(jīng)構(gòu)建出實(shí)驗(yàn)所需的數(shù)據(jù):
訓(xùn)練數(shù)據(jù)(這里說的是dataset_train.csv而不是train.csv)
驗(yàn)證數(shù)據(jù)(dataset_train.csv)
測試數(shù)據(jù)(test.csv)。
二、分詞
導(dǎo)入的數(shù)據(jù)是字符串形式的文本,我們需要將其分詞成詞語列表。英文最精準(zhǔn)的分詞器如下:
import re
import spacy
import jieba
#英文的分詞器
NLP = spacy.load('en_core_web_sm')
MAX_CHARS = 20000 #為了降低處理的數(shù)據(jù)規(guī)模,可以設(shè)置最大文本長度,超過的部分忽略,
def tokenize1(text):
text = re.sub(r"\s", " ", text)
if (len(text) gt; MAX_CHARS):
text = text[:MAX_CHARS]
return [
x.text for x in NLP.tokenizer(text) if x.text != " " and len(x.text)gt;1]
#有的同學(xué)tokenize1用不了,可以使用tokenize2。
def tokenize2(text):
text = re.sub(r"\s", " ", text)
if (len(text) gt; MAX_CHARS):
text = text[:MAX_CHARS]
return [w for w in text.split(' ') if len(w)gt;1]
#中文的分類器比較簡單
def tokenize3(text):
if (len(text) gt; MAX_CHARS):
text = text[:MAX_CHARS]
return [w for w in jieba.lcut(text) if len(w)gt;1]
print(tokenize1('Python is powerful and beautiful!'))
print(tokenize2('Python is powerful and beautiful!'))
print(tokenize3('Python強(qiáng)大而美麗!'))
Run
['Python', 'is', 'powerful', 'and', 'beautiful']
['Python', 'is', 'powerful', 'and', 'beautiful!']
['Python', '強(qiáng)大', '美麗']
三、 導(dǎo)入數(shù)據(jù)
torchtext中使用torchtext.data.TabularDataset來導(dǎo)入自己的數(shù)據(jù)集,并且我們需要先定義字段的數(shù)據(jù)類型才能導(dǎo)入。要按照csv中的字段順序來定義字段的數(shù)據(jù)類型,我們的csv文件中有兩個(gè)字段(label、text)
import pandas as pd
df = pd.read_csv('data/train.csv')
df.head()
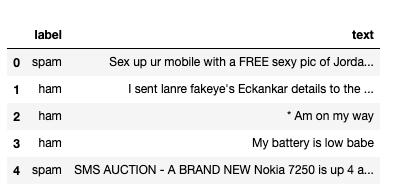
import torch
import torchtext
from torchtext import data
import logging
LABEL = data.LabelField(dtype = torch.float)
TEXT = data.Field(tokenize = tokenize1,
lower=True,
fix_length=100,
stop_words=None)
train, valid, test = data.TabularDataset.splits(path='data', #數(shù)據(jù)所在文件夾
train='dataset_train.csv',
validation='dataset_valid.csv',
test = 'test.csv',
format='csv',
skip_header=True,
fields = [('label', LABEL),('text', TEXT)])
train
Run
lt;torchtext.data.dataset.TabularDataset at 0x120d8ab38gt;
四、構(gòu)建詞典
根據(jù)訓(xùn)練(上面得到的train)的預(yù)料數(shù)據(jù)集構(gòu)建詞典。這兩有兩種構(gòu)建方式,一種是常規(guī)的不使用詞向量,而另一種是使用向量的。
區(qū)別僅僅在于vectors是否傳入?yún)?shù)
vects = torchtext.vocab.Vectors(name = 'glove.6B.100d.txt',
cache = 'data/')
TEXT.build_vocab(train,
max_size=2000,
min_freq=50,
vectors=vects, #vects替換為None則不使用詞向量
unk_init = torch.Tensor.normal_)
4.1 TEXT是Field對象,該對象的方法有
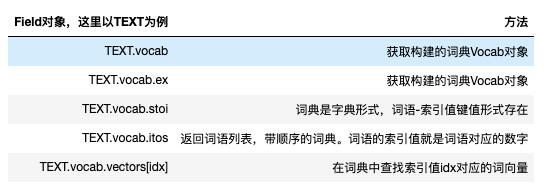
print(type(TEXT))
print(type(TEXT.vocab))
Run
lt;class 'torchtext.data.field.Field'gt;
lt;class 'torchtext.vocab.Vocab'gt;
詞典-詞語列表形式,這里只顯示前20個(gè)
['lt;unkgt;',
'lt;padgt;',
'to',
'you',
'the',
'...',
'and',
'is',
'in',
'me',
'it',
'my',
'for',
'your',
'..',
'do',
'of',
'have',
'that',
'call']
詞典-字典形式
defaultdict(lt;bound method Vocab._default_unk_index of lt;torchtext.vocab.Vocab object at 0x1214b1e48gt;gt;,
{'lt;unkgt;': 0,
'lt;padgt;': 1,
'to': 2,
'you': 3,
'the': 4,
'...': 5,
'and': 6,
'is': 7,
'in': 8,
....
'mother': 0,
'english': 0,
'son': 0,
'gradfather': 0,
'father': 0,
'german': 0)
4.2 注意
train數(shù)據(jù)中生成的詞典,里面有,這里有兩個(gè)要注意:
是指不認(rèn)識的詞語都編碼為
german、father等都編碼為0,這是因?yàn)槲覀円笤~典中出現(xiàn)的詞語詞頻必須大于50,小于50的都統(tǒng)一分配一個(gè)索引值。
詞語you對應(yīng)的詞向量
tensor([-0.4989, 0.7660, 0.8975, -0.7855, -0.6855, 0.6261, -0.3965, 0.3491,
0.3333, -0.4523, 0.6122, 0.0759, 0.2253, 0.1637, 0.2810, -0.2476,
0.0099, 0.7111, -0.7586, 0.8742, 0.0031, 0.3580, -0.3523, -0.6650,
0.3845, 0.6268, -0.5154, -0.9665, 0.6152, -0.7545, -0.0124, 1.1188,
0.3572, 0.0072, 0.2025, 0.5011, -0.4405, 0.1066, 0.7939, -0.8095,
-0.0156, -0.2289, -0.3420, -1.0065, -0.8763, 0.1516, -0.0853, -0.6465,
-0.1673, -1.4499, -0.0066, 0.0048, -0.0124, 1.0474, -0.1938, -2.5991,
0.4053, 0.4380, 1.9332, 0.4581, -0.0488, 1.4308, -0.7864, -0.2079,
1.0900, 0.2482, 1.1487, 0.5148, -0.2183, -0.4572, 0.1389, -0.2637,
0.1365, -0.6054, 0.0996, 0.2334, 0.1365, -0.1846, -0.0477, -0.1839,
0.5272, -0.2885, -1.0742, -0.0467, -1.8302, -0.2120, 0.0298, -0.3096,
-0.4339, -0.3646, -0.3274, -0.0093, 0.4721, -0.5169, -0.5918, -0.3234,
0.2005, -0.4118, 0.4054, 0.7850])
4.3 計(jì)算詞語的相似性
得用詞向量構(gòu)建特征工程時(shí)能保留更多的信息量(詞語之間的關(guān)系)
這樣可以看出詞語的向量方向
是同義還是反義
距離遠(yuǎn)近。
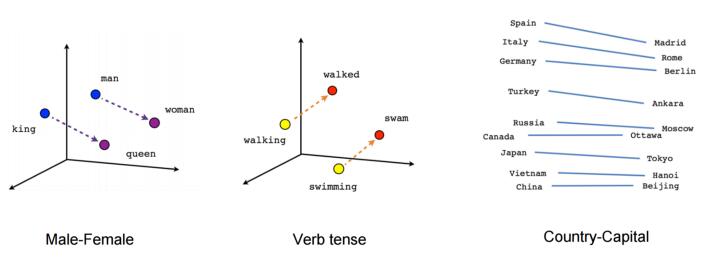
而這里我們粗糙的用余弦定理計(jì)算詞語之間的關(guān)系,沒有近義反義關(guān)系,只能體現(xiàn)出距離遠(yuǎn)近(相似性)。
from sklearn.metrics.pairwise import cosine_similarity
import numpy as np
def simalarity(word1, word2):
word_vec1 = TEXT.vocab.vectors[TEXT.vocab.stoi[word1]].tolist()
word_vec2 = TEXT.vocab.vectors[TEXT.vocab.stoi[word2]].tolist()
vectors = np.array([word_vec1, word_vec2])
return cosine_similarity(vectors)
print(simalarity('you', 'your'))
Run
[[1. 0.83483314]
[0.83483314 1. ]]
五、get_dataset函數(shù)
相似的功能合并成模塊,可以增加代碼的可讀性。這里我們把階段性合并三四的成果get_dataset函數(shù)
from torchtext import data
import torchtext
import torch
import logging
LOGGER = logging.getLogger("導(dǎo)入數(shù)據(jù)")
def get_dataset(stop_words=None):
#定義字段的數(shù)據(jù)類型
LABEL = data.LabelField(dtype = torch.float)
TEXT = data.Field(tokenize = tokenize1,
lower=True,
fix_length=100,
stop_words=stop_words)
LOGGER.debug("準(zhǔn)備讀取csv數(shù)據(jù)...")
train, valid, test = data.TabularDataset.splits(path='data', #數(shù)據(jù)所在文件夾
train='dataset_train.csv',
validation='dataset_valid.csv',
test = 'test.csv',
format='csv',
skip_header=True,
fields = [('label', LABEL),('text', TEXT)])
LOGGER.debug("準(zhǔn)備導(dǎo)入詞向量...")
vectors = torchtext.vocab.Vectors(name = 'glove.6B.100d.txt',
cache = 'data/')
LOGGER.debug("準(zhǔn)備構(gòu)建詞典...")
TEXT.build_vocab(
train,
max_size=2000,
min_freq=50,
vectors=vectors,
unk_init = torch.Tensor.normal_)
LOGGER.debug("完成數(shù)據(jù)導(dǎo)入!")
return train,valid, test, TEXT
get_dataset函數(shù)內(nèi)部參數(shù)解讀
data.Field(tokenize,fix_length)定義字段
tokenize=tokenize1 使用英文的分詞器tokenize1函數(shù)。
fix_length=100 讓每個(gè)文本分詞后的長度均為100個(gè)詞;不足100的,可以填充為100。超過100的,只保留100
data.TabularDataset.splits(train, validation,test, format,skip_header,fields)讀取訓(xùn)練驗(yàn)證數(shù)據(jù),可以一次性讀取多個(gè)文件
train/validation/test 訓(xùn)練驗(yàn)證測試對應(yīng)的csv文件名
skip_header=True 如果csv有抬頭,設(shè)置為True可以避免pytorch將抬頭當(dāng)成一條記錄
fields = [('label', LABEL), ('text', TEXT)] 定義字段的類型,注意fields要按照csv抬頭中字段的順序設(shè)置
torchtext.vocab.Vectors(name, cache)導(dǎo)入詞向量數(shù)據(jù)文件
name= 'glove.6B.100d.txt' 從網(wǎng)上下載預(yù)訓(xùn)練好的詞向量glove.6B.100d.txt文件(該文件有6B個(gè)詞,每個(gè)詞向量長度為100)
cache = 'data/' 文件夾位置。glove文件存放在data文件夾內(nèi)
TEXT.buildvocab(maxsize,minfreq,unkinit) 構(gòu)建詞典,其中
max_size=2000 設(shè)定了詞典最大詞語數(shù)
min_freq=50設(shè)定了詞典中的詞語保證最少出現(xiàn)50次
unkinit=torch.Tensor.normal 詞典中沒有的詞語對應(yīng)的向量統(tǒng)一用torch.Tensor.normal_填充
六、分批次
數(shù)據(jù)集太大的話,一次性讓機(jī)器讀取容易導(dǎo)致內(nèi)存崩潰。解決辦法就是將大的數(shù)據(jù)集分成更小份的數(shù)據(jù)集,分批處理
def split2batches(batch_size=32, device='cpu'):
train, valid, test, TEXT = get_dataset() #datasets按順序包含train、valid、test三部分
LOGGER.debug("準(zhǔn)備數(shù)據(jù)分批次...")
train_iterator, valid_iterator, test_iterator = data.BucketIterator.splits((train, valid, test),
batch_size = batch_size,
sort = False,
device = device)
LOGGER.debug("完成數(shù)據(jù)分批次!")
return train_iterator, valid_iterator, test_iterator, TEXT
6.1參數(shù)解讀
split2batches(batch_size=32, device=0)
batch_size 每批次最多加入多少條評論
device device='cpu'在CPU中運(yùn)行,device='gpu' 在GPU中運(yùn)行。普通電腦都只有CPU的 該函數(shù)返回的是BucketIterator對象
train_iterator, valid_iterator, test_iterator, TEXT = split2batches()
train_iterator
Run
lt;torchtext.data.iterator.BucketIterator at 0x12b0c7898gt;
查看train_iterator數(shù)據(jù)類型
type(train_iterator)
torchtext.data.iterator.BucketIterator
6.2BucketIterator對象
這里以trainiterator為例(validiterator, test_iterator都是相同的對象)。因?yàn)楸纠袛?shù)據(jù)有兩個(gè)字段label和text,所以
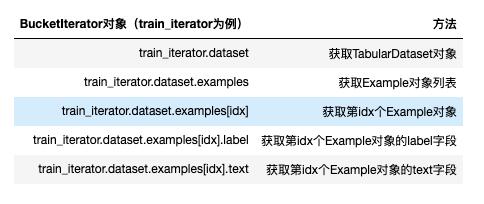
獲取train_iterator的dataset
train_iterator.dataset
lt;torchtext.data.dataset.TabularDataset at 0x12e9c57b8gt;
獲取train_iterator中的第8個(gè)對象
train_iterator.dataset.examples[7]
lt;torchtext.data.example.Example at 0x12a82dcf8gt;
獲取train_iterator中的第8個(gè)對象的lebel字段的內(nèi)容
train_iterator.dataset.examples[7].label
'ham'
獲取train_iterator中的第8個(gè)對象的text字段的內(nèi)容
train_iterator.dataset.examples[7].text
['were', 'trying', 'to', 'find', 'chinese', 'food', 'place', 'around', 'here']
總結(jié)
到這里我們已經(jīng)學(xué)習(xí)了torchtext的常用知識。使用本代碼要注意:
我們假設(shè)數(shù)據(jù)集是csv文件,torchtext可以還可以處理tsv、json。但如果你想使用本代碼,請先轉(zhuǎn)為csv
本教程的csv文件只有兩個(gè)字段,label和text。如果你的數(shù)據(jù)有更多的字段,記得再代碼中增加字段定義
本教程默認(rèn)場景是英文,且使用詞向量。所以記得對應(yīng)位置下載本教程的glove.6B.100d.txt。
glove下載地址https://nlp.stanford.edu/projects/glove/
以上為個(gè)人經(jīng)驗(yàn),希望能給大家一個(gè)參考,也希望大家多多支持腳本之家。
您可能感興趣的文章:- 使用pytorch和torchtext進(jìn)行文本分類的實(shí)例
- pytorch加載語音類自定義數(shù)據(jù)集的方法教程
- pytorch下大型數(shù)據(jù)集(大型圖片)的導(dǎo)入方式
- PyTorch加載自己的數(shù)據(jù)集實(shí)例詳解