DBSCAN 算法是一種基于密度的空間聚類算法。該算法利用基于密度的聚類的概念,即要求聚類空間中的一定區(qū)域內所包含對象(點或其它空間對象)的數目不小于某一給定閥值。DBSCAN 算法的顯著優(yōu)點是聚類速度快且能夠有效處理噪聲點和發(fā)現任意形狀的空間聚類。但是由于它直接對整個數據庫進行操作且進行聚類時使用了一個全局性的表征密度的參數,因此也具有兩個比較明顯的弱點:
1. 當數據量增大時,要求較大的內存支持 I/0 消耗也很大;
2. 當空間聚類的密度不均勻、聚類間距離相差很大時,聚類質量較差。
DBSCAN算法的聚類過程
DBSCAN算法基于一個事實:一個聚類可以由其中的任何核心對象唯一確定。等價可以表述為: 任一滿足核心對象條件的數據對象p,數據庫D中所有從p密度可達的數據對象所組成的集合構成了一個完整的聚類C,且p屬于C。
先上結果
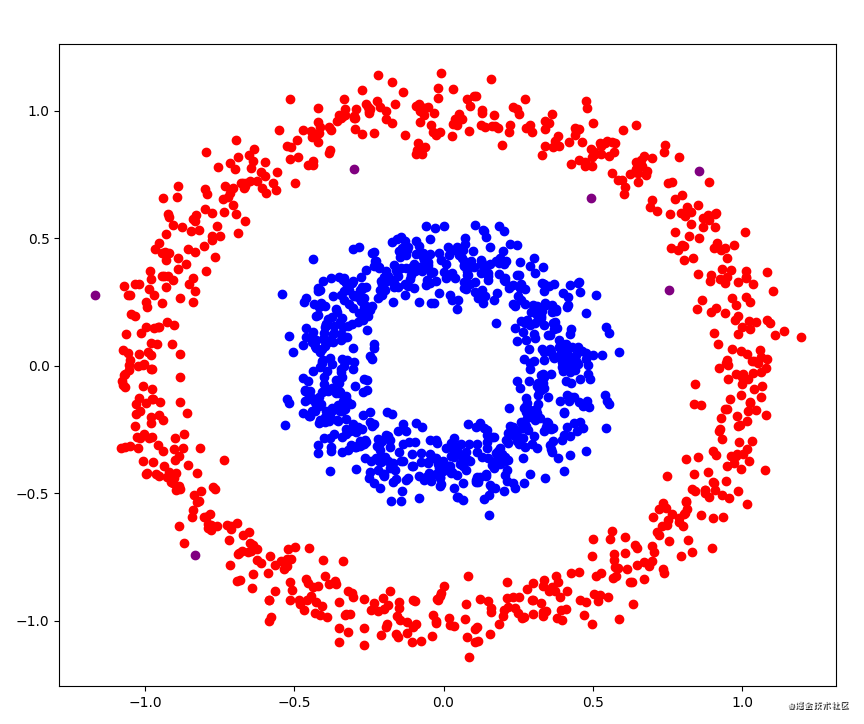
大致流程
先根據給定的半徑 r 確定中心點,也就是這類點在半徑r內包含的點數量 n 大于我們的要求(n>=minPionts)
然后遍歷所有的中心點,將互相可通達的中心點與其包括的點分為一組
全部分完組之后,沒有被納入任何一組的點就是離群點啦!
導入相關依賴
import numpy as np
import matplotlib.pyplot as plt
from sklearn import datasets
求點跟點之間距離(歐氏距離)
def cuircl(pointA,pointB):
distance = np.sqrt(np.sum(np.power(pointA - pointB,2)))
return distance
求臨時簇,即確定所有的中心點,非中心點
def firstCluster(dataSets,r,include):
cluster = []
m = np.shape(dataSets)[0]
ungrouped = np.array([i for i in range (m)])
for i in range (m):
tempCluster = []
#第一位存儲中心點簇
tempCluster.append(i)
for j in range (m):
if (cuircl(dataSets[i,:],dataSets[j,:]) r and i != j ):
tempCluster.append(j)
tempCluster = np.mat(np.array(tempCluster))
if (np.size(tempCluster)) >= include:
cluster.append(np.array(tempCluster).flatten())
#返回的是List
center=[]
n = np.shape(cluster)[0]
for k in range (n):
center.append(cluster[k][0])
#其他的就是非中心點啦
ungrouped = np.delete(ungrouped,center)
#ungrouped為非中心點
return cluster,center,ungrouped
將所有中心點遍歷并進行聚集
def clusterGrouped(tempcluster,centers):
m = np.shape(tempcluster)[0]
group = []
#對應點是否遍歷過
position = np.ones(m)
unvisited = []
#未遍歷點
unvisited.extend(centers)
#所有點均遍歷完畢
for i in range (len(position)):
coreNeihbor = []
result = []
#刪除第一個
#刨去自己的鄰居結點,這一段就類似于深度遍歷
if position[i]:
#將鄰結點填入
coreNeihbor.extend(list(tempcluster[i][:]))
position[i] = 0
temp = coreNeihbor
#按照深度遍歷遍歷完所有可達點
#遍歷完所有的鄰居結點
while len(coreNeihbor) > 0 :
#選擇當前點
present = coreNeihbor[0]
for j in range(len(position)):
#如果沒有訪問過
if position[j] == 1:
same = []
#求所有的可達點
if (present in tempcluster[j]):
cluster = tempcluster[j].tolist()
diff = []
for x in cluster:
if x not in temp:
#確保沒有重復點
diff.append(x)
temp.extend(diff)
position[j] = 0
# 刪掉當前點
del coreNeihbor[0]
result.extend(temp)
group.append(list(set(result)))
i +=1
return group
核心算法完畢!
生成同心圓類型的隨機數據進行測試
#生成非凸數據 factor表示內外圈距離比
X,Y1 = datasets.make_circles(n_samples = 1500, factor = .4, noise = .07)
#參數選擇,0.1為圓半徑,6為判定中心點所要求的點個數,生成分類結果
tempcluster,center,ungrouped = firstCluster(X,0.1,6)
group = clusterGrouped(tempcluster,center)
#以下是分類后對數據進行進一步處理
num = len(group)
voice = list(ungrouped)
Y = []
for i in range (num):
Y.append(X[group[i]])
flat = []
for i in range(num):
flat.extend(group[i])
diff = [x for x in voice if x not in flat]
Y.append(X[diff])
Y = np.mat(np.array(Y))
繪圖~
color = ['red','blue','green','black','pink','orange']
for i in range(num):
plt.scatter(Y[0,i][:,0],Y[0,i][:,1],c=color[i])
plt.scatter(Y[0,-1][:,0],Y[0,-1][:,1],c = 'purple')
plt.show()
結果
紫色點就是離散點
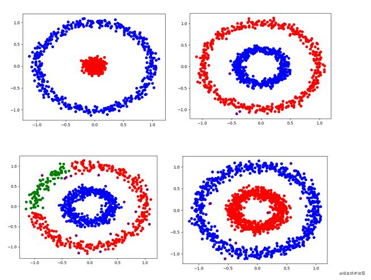
到此這篇關于python實現dbscan算法的文章就介紹到這了,更多相關python dbscan算法內容請搜索腳本之家以前的文章或繼續(xù)瀏覽下面的相關文章希望大家以后多多支持腳本之家!
您可能感興趣的文章:- python實現鳶尾花三種聚類算法(K-means,AGNES,DBScan)
- Python基于聚類算法實現密度聚類(DBSCAN)計算【測試可用】
- K-means聚類算法介紹與利用python實現的代碼示例
- python中實現k-means聚類算法詳解
- Python聚類算法之DBSACN實例分析
- Python實現DBSCAN聚類算法并樣例測試