目錄
- 簡介
- 使用concat
- 使用append
- 使用merge
- 使用join
- 覆蓋數(shù)據(jù)
簡介
Pandas提供了很多合并Series和Dataframe的強大的功能,通過這些功能可以方便的進(jìn)行數(shù)據(jù)分析。本文將會詳細(xì)講解如何使用Pandas來合并Series和Dataframe。
使用concat
concat是最常用的合并DF的方法,先看下concat的定義:
pd.concat(objs, axis=0, join='outer', ignore_index=False, keys=None,
levels=None, names=None, verify_integrity=False, copy=True)
看一下我們經(jīng)常會用到的幾個參數(shù):
objs是Series或者Series的序列或者映射。
axis指定連接的軸。
join
: {‘inner', ‘outer'}, 連接方式,怎么處理其他軸的index,outer表示合并,inner表示交集。
ignore_index: 忽略原本的index值,使用0,1,… n-1來代替。
copy:是否進(jìn)行拷貝。
keys:指定最外層的多層次結(jié)構(gòu)的index。
我們先定義幾個DF,然后看一下怎么使用concat把這幾個DF連接起來:
In [1]: df1 = pd.DataFrame({'A': ['A0', 'A1', 'A2', 'A3'],
...: 'B': ['B0', 'B1', 'B2', 'B3'],
...: 'C': ['C0', 'C1', 'C2', 'C3'],
...: 'D': ['D0', 'D1', 'D2', 'D3']},
...: index=[0, 1, 2, 3])
...:
In [2]: df2 = pd.DataFrame({'A': ['A4', 'A5', 'A6', 'A7'],
...: 'B': ['B4', 'B5', 'B6', 'B7'],
...: 'C': ['C4', 'C5', 'C6', 'C7'],
...: 'D': ['D4', 'D5', 'D6', 'D7']},
...: index=[4, 5, 6, 7])
...:
In [3]: df3 = pd.DataFrame({'A': ['A8', 'A9', 'A10', 'A11'],
...: 'B': ['B8', 'B9', 'B10', 'B11'],
...: 'C': ['C8', 'C9', 'C10', 'C11'],
...: 'D': ['D8', 'D9', 'D10', 'D11']},
...: index=[8, 9, 10, 11])
...:
In [4]: frames = [df1, df2, df3]
In [5]: result = pd.concat(frames)
df1,df2,df3定義了同樣的列名和不同的index,然后將他們放在frames中構(gòu)成了一個DF的list,將其作為參數(shù)傳入concat就可以進(jìn)行DF的合并。
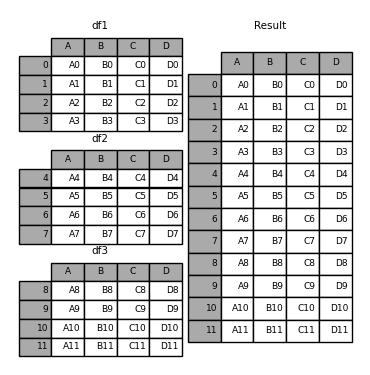
舉個多層級的例子:
In [6]: result = pd.concat(frames, keys=['x', 'y', 'z'])
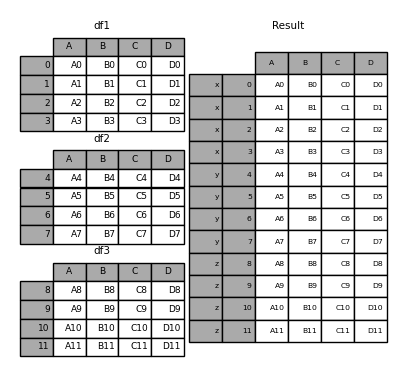
使用keys可以指定frames中不同frames的key。
使用的時候,我們可以通過選擇外部的key來返回特定的frame:
In [7]: result.loc['y']
Out[7]:
A B C D
4 A4 B4 C4 D4
5 A5 B5 C5 D5
6 A6 B6 C6 D6
7 A7 B7 C7 D7
上面的例子連接的軸默認(rèn)是0,也就是按行來進(jìn)行連接,下面我們來看一個例子按列來進(jìn)行連接,如果要按列來連接,可以指定axis=1:
In [8]: df4 = pd.DataFrame({'B': ['B2', 'B3', 'B6', 'B7'],
...: 'D': ['D2', 'D3', 'D6', 'D7'],
...: 'F': ['F2', 'F3', 'F6', 'F7']},
...: index=[2, 3, 6, 7])
...:
In [9]: result = pd.concat([df1, df4], axis=1, sort=False)
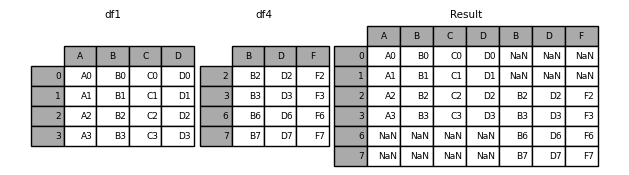
默認(rèn)的 join='outer'
,合并之后index不存在的地方會補全為NaN。
下面看一個join='inner'的情況:
In [10]: result = pd.concat([df1, df4], axis=1, join='inner')

join='inner' 只會選擇index相同的進(jìn)行展示。
如果合并之后,我們只想保存原來frame的index相關(guān)的數(shù)據(jù),那么可以使用reindex:
In [11]: result = pd.concat([df1, df4], axis=1).reindex(df1.index)
或者這樣:
In [12]: pd.concat([df1, df4.reindex(df1.index)], axis=1)
Out[12]:
A B C D B D F
0 A0 B0 C0 D0 NaN NaN NaN
1 A1 B1 C1 D1 NaN NaN NaN
2 A2 B2 C2 D2 B2 D2 F2
3 A3 B3 C3 D3 B3 D3 F3
看下結(jié)果:

可以合并DF和Series:
In [18]: s1 = pd.Series(['X0', 'X1', 'X2', 'X3'], name='X')
In [19]: result = pd.concat([df1, s1], axis=1)
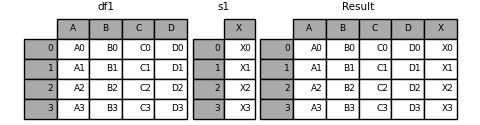
如果是多個Series,使用concat可以指定列名:
In [23]: s3 = pd.Series([0, 1, 2, 3], name='foo')
In [24]: s4 = pd.Series([0, 1, 2, 3])
In [25]: s5 = pd.Series([0, 1, 4, 5])
In [27]: pd.concat([s3, s4, s5], axis=1, keys=['red', 'blue', 'yellow'])
Out[27]:
red blue yellow
0 0 0 0
1 1 1 1
2 2 2 4
3 3 3 5
使用append
append可以看做是concat的簡化版本,它沿著axis=0
進(jìn)行concat:
In [13]: result = df1.append(df2)
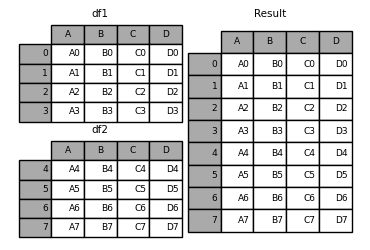
如果append的兩個 DF的列是不一樣的會自動補全NaN:
In [14]: result = df1.append(df4, sort=False)
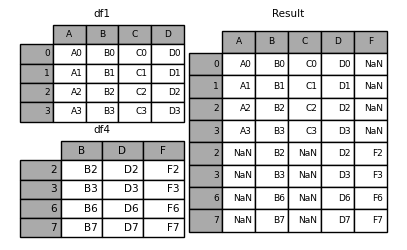
如果設(shè)置ignore_index=True,可以忽略原來的index,并重寫分配index:
In [17]: result = df1.append(df4, ignore_index=True, sort=False)

向DF append一個Series:
In [35]: s2 = pd.Series(['X0', 'X1', 'X2', 'X3'], index=['A', 'B', 'C', 'D'])
In [36]: result = df1.append(s2, ignore_index=True)

使用merge
和DF最類似的就是數(shù)據(jù)庫的表格,可以使用merge來進(jìn)行類似數(shù)據(jù)庫操作的DF合并操作。
先看下merge的定義:
pd.merge(left, right, how='inner', on=None, left_on=None, right_on=None,
left_index=False, right_index=False, sort=True,
suffixes=('_x', '_y'), copy=True, indicator=False,
validate=None)
Left, right是要合并的兩個DF 或者 Series。
on代表的是join的列或者index名。
left_on:左連接
right_on
:右連接
left_index
: 連接之后,選擇使用左邊的index或者column。
right_index
:連接之后,選擇使用右邊的index或者column。
how:連接的方式,'left'
, 'right'
, 'outer'
, 'inner'
. 默認(rèn) inner
.
sort
: 是否排序。
suffixes
: 處理重復(fù)的列。
copy
: 是否拷貝數(shù)據(jù)
先看一個簡單merge的例子:
In [39]: left = pd.DataFrame({'key': ['K0', 'K1', 'K2', 'K3'],
....: 'A': ['A0', 'A1', 'A2', 'A3'],
....: 'B': ['B0', 'B1', 'B2', 'B3']})
....:
In [40]: right = pd.DataFrame({'key': ['K0', 'K1', 'K2', 'K3'],
....: 'C': ['C0', 'C1', 'C2', 'C3'],
....: 'D': ['D0', 'D1', 'D2', 'D3']})
....:
In [41]: result = pd.merge(left, right, on='key')
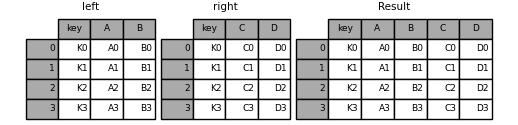
上面兩個DF通過key來進(jìn)行連接。
再看一個多個key連接的例子:
In [42]: left = pd.DataFrame({'key1': ['K0', 'K0', 'K1', 'K2'],
....: 'key2': ['K0', 'K1', 'K0', 'K1'],
....: 'A': ['A0', 'A1', 'A2', 'A3'],
....: 'B': ['B0', 'B1', 'B2', 'B3']})
....:
In [43]: right = pd.DataFrame({'key1': ['K0', 'K1', 'K1', 'K2'],
....: 'key2': ['K0', 'K0', 'K0', 'K0'],
....: 'C': ['C0', 'C1', 'C2', 'C3'],
....: 'D': ['D0', 'D1', 'D2', 'D3']})
....:
In [44]: result = pd.merge(left, right, on=['key1', 'key2'])

How 可以指定merge方式,和數(shù)據(jù)庫一樣,可以指定是內(nèi)連接,外連接等:
合并方法 |
SQL 方法 |
left |
LEFT OUTER JOIN |
right |
RIGHT OUTER JOIN |
outer |
FULL OUTER JOIN |
inner |
INNER JOIN |
|
In [45]: result = pd.merge(left, right, how='left', on=['key1', 'key2'])
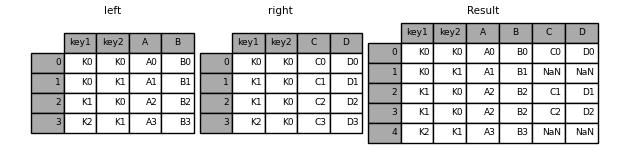
指定indicator=True ,可以表示具體行的連接方式:
In [60]: df1 = pd.DataFrame({'col1': [0, 1], 'col_left': ['a', 'b']})
In [61]: df2 = pd.DataFrame({'col1': [1, 2, 2], 'col_right': [2, 2, 2]})
In [62]: pd.merge(df1, df2, on='col1', how='outer', indicator=True)
Out[62]:
col1 col_left col_right _merge
0 0 a NaN left_only
1 1 b 2.0 both
2 2 NaN 2.0 right_only
3 2 NaN 2.0 right_only
如果傳入字符串給indicator,會重命名indicator這一列的名字:
In [63]: pd.merge(df1, df2, on='col1', how='outer', indicator='indicator_column')
Out[63]:
col1 col_left col_right indicator_column
0 0 a NaN left_only
1 1 b 2.0 both
2 2 NaN 2.0 right_only
3 2 NaN 2.0 right_only
多個index進(jìn)行合并:
In [112]: leftindex = pd.MultiIndex.from_tuples([('K0', 'X0'), ('K0', 'X1'),
.....: ('K1', 'X2')],
.....: names=['key', 'X'])
.....:
In [113]: left = pd.DataFrame({'A': ['A0', 'A1', 'A2'],
.....: 'B': ['B0', 'B1', 'B2']},
.....: index=leftindex)
.....:
In [114]: rightindex = pd.MultiIndex.from_tuples([('K0', 'Y0'), ('K1', 'Y1'),
.....: ('K2', 'Y2'), ('K2', 'Y3')],
.....: names=['key', 'Y'])
.....:
In [115]: right = pd.DataFrame({'C': ['C0', 'C1', 'C2', 'C3'],
.....: 'D': ['D0', 'D1', 'D2', 'D3']},
.....: index=rightindex)
.....:
In [116]: result = pd.merge(left.reset_index(), right.reset_index(),
.....: on=['key'], how='inner').set_index(['key', 'X', 'Y'])
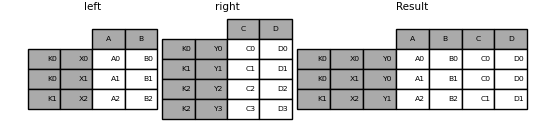
支持多個列的合并:
In [117]: left_index = pd.Index(['K0', 'K0', 'K1', 'K2'], name='key1')
In [118]: left = pd.DataFrame({'A': ['A0', 'A1', 'A2', 'A3'],
.....: 'B': ['B0', 'B1', 'B2', 'B3'],
.....: 'key2': ['K0', 'K1', 'K0', 'K1']},
.....: index=left_index)
.....:
In [119]: right_index = pd.Index(['K0', 'K1', 'K2', 'K2'], name='key1')
In [120]: right = pd.DataFrame({'C': ['C0', 'C1', 'C2', 'C3'],
.....: 'D': ['D0', 'D1', 'D2', 'D3'],
.....: 'key2': ['K0', 'K0', 'K0', 'K1']},
.....: index=right_index)
.....:
In [121]: result = left.merge(right, on=['key1', 'key2'])
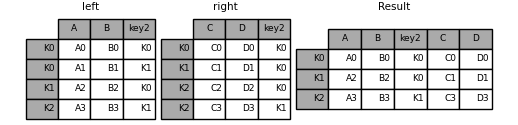
使用join
join將兩個不同index的DF合并成一個。可以看做是merge的簡寫。
In [84]: left = pd.DataFrame({'A': ['A0', 'A1', 'A2'],
....: 'B': ['B0', 'B1', 'B2']},
....: index=['K0', 'K1', 'K2'])
....:
In [85]: right = pd.DataFrame({'C': ['C0', 'C2', 'C3'],
....: 'D': ['D0', 'D2', 'D3']},
....: index=['K0', 'K2', 'K3'])
....:
In [86]: result = left.join(right)
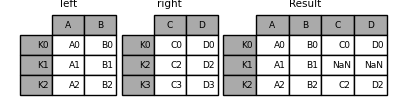
可以指定how來指定連接方式:
In [87]: result = left.join(right, how='outer')
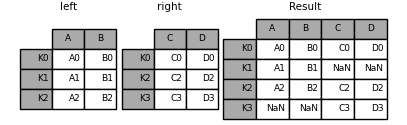
默認(rèn)join是按index來進(jìn)行連接。
還可以按照列來進(jìn)行連接:
In [91]: left = pd.DataFrame({'A': ['A0', 'A1', 'A2', 'A3'],
....: 'B': ['B0', 'B1', 'B2', 'B3'],
....: 'key': ['K0', 'K1', 'K0', 'K1']})
....:
In [92]: right = pd.DataFrame({'C': ['C0', 'C1'],
....: 'D': ['D0', 'D1']},
....: index=['K0', 'K1'])
....:
In [93]: result = left.join(right, on='key')
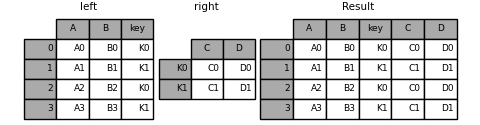
單個index和多個index進(jìn)行join:
In [100]: left = pd.DataFrame({'A': ['A0', 'A1', 'A2'],
.....: 'B': ['B0', 'B1', 'B2']},
.....: index=pd.Index(['K0', 'K1', 'K2'], name='key'))
.....:
In [101]: index = pd.MultiIndex.from_tuples([('K0', 'Y0'), ('K1', 'Y1'),
.....: ('K2', 'Y2'), ('K2', 'Y3')],
.....: names=['key', 'Y'])
.....:
In [102]: right = pd.DataFrame({'C': ['C0', 'C1', 'C2', 'C3'],
.....: 'D': ['D0', 'D1', 'D2', 'D3']},
.....: index=index)
.....:
In [103]: result = left.join(right, how='inner')
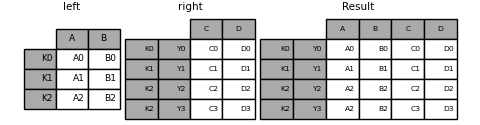
列名重復(fù)的情況:
In [122]: left = pd.DataFrame({'k': ['K0', 'K1', 'K2'], 'v': [1, 2, 3]})
In [123]: right = pd.DataFrame({'k': ['K0', 'K0', 'K3'], 'v': [4, 5, 6]})
In [124]: result = pd.merge(left, right, on='k')
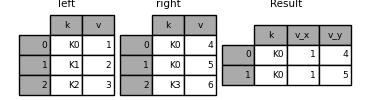
可以自定義重復(fù)列名的命名規(guī)則:
In [125]: result = pd.merge(left, right, on='k', suffixes=('_l', '_r'))
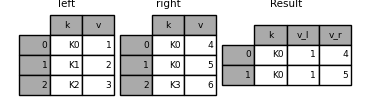
覆蓋數(shù)據(jù)
有時候我們需要使用DF2的數(shù)據(jù)來填充DF1的數(shù)據(jù),這時候可以使用combine_first:
In [131]: df1 = pd.DataFrame([[np.nan, 3., 5.], [-4.6, np.nan, np.nan],
.....: [np.nan, 7., np.nan]])
.....:
In [132]: df2 = pd.DataFrame([[-42.6, np.nan, -8.2], [-5., 1.6, 4]],
.....: index=[1, 2])
.....:
In [133]: result = df1.combine_first(df2)
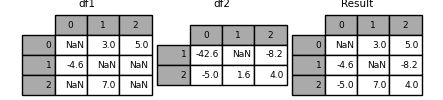
到此這篇關(guān)于Pandas實現(xiàn)Dataframe的合并的文章就介紹到這了,更多相關(guān)Pandas Dataframe合并內(nèi)容請搜索腳本之家以前的文章或繼續(xù)瀏覽下面的相關(guān)文章希望大家以后多多支持腳本之家!
您可能感興趣的文章:- Pandas實現(xiàn)Dataframe的重排和旋轉(zhuǎn)
- pandas中DataFrame數(shù)據(jù)合并連接(merge、join、concat)
- 教你漂亮打印Pandas DataFrames和Series
- pandas中DataFrame檢測重復(fù)值的實現(xiàn)
- 使用pandas忽略行列索引,縱向拼接多個dataframe
- Pandas.DataFrame轉(zhuǎn)置的實現(xiàn)
- Pandas中DataFrame交換列順序的方法實現(xiàn)
- 詳解pandas中利用DataFrame對象的.loc[]、.iloc[]方法抽取數(shù)據(jù)
- Pandas中兩個dataframe的交集和差集的示例代碼
- Pandas DataFrame求差集的示例代碼
- 淺談pandas dataframe對除數(shù)是零的處理
- Pandas中DataFrame數(shù)據(jù)刪除詳情