目錄
- 概述
- Sequential
- Model Layer
- 案例
概述
通過自定義網(wǎng)絡(luò), 我們可以自己創(chuàng)建網(wǎng)絡(luò)并和現(xiàn)有的網(wǎng)絡(luò)串聯(lián)起來, 從而實現(xiàn)各種各樣的網(wǎng)絡(luò)結(jié)構(gòu).
Sequential
Sequential 是 Keras 的一個網(wǎng)絡(luò)容器. 可以幫助我們將多層網(wǎng)絡(luò)封裝在一起.
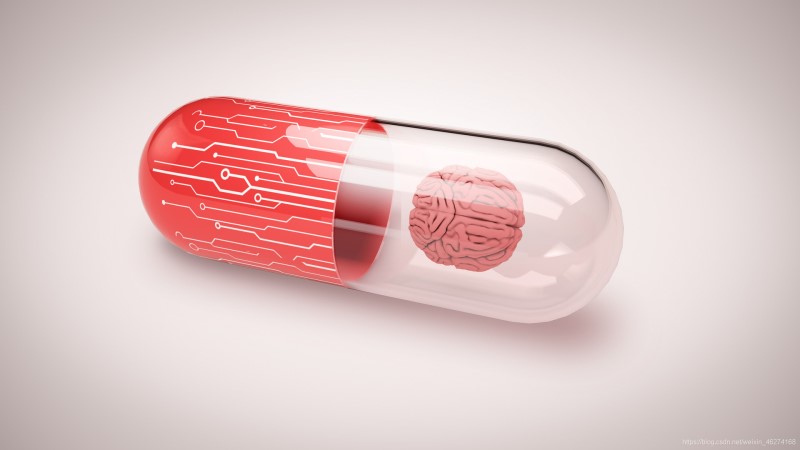
通過 Sequential 我們可以把現(xiàn)有的層已經(jīng)我們自己的層實現(xiàn)結(jié)合, 一次前向傳播就可以實現(xiàn)數(shù)據(jù)從第一層到最后一層的計算.
格式:
tf.keras.Sequential(
layers=None, name=None
)
例子:
# 5層網(wǎng)絡(luò)模型
model = tf.keras.Sequential([
tf.keras.layers.Dense(256, activation=tf.nn.relu),
tf.keras.layers.Dense(128, activation=tf.nn.relu),
tf.keras.layers.Dense(64, activation=tf.nn.relu),
tf.keras.layers.Dense(32, activation=tf.nn.relu),
tf.keras.layers.Dense(10)
])
Model Layer
通過 Model 和 Layer 的__init__
和call()
我們可以自定義層和模型.
Model:
class My_Model(tf.keras.Model): # 繼承Model
def __init__(self):
"""
初始化
"""
super(My_Model, self).__init__()
self.fc1 = My_Dense(784, 256) # 第一層
self.fc2 = My_Dense(256, 128) # 第二層
self.fc3 = My_Dense(128, 64) # 第三層
self.fc4 = My_Dense(64, 32) # 第四層
self.fc5 = My_Dense(32, 10) # 第五層
def call(self, inputs, training=None):
"""
在Model被調(diào)用的時候執(zhí)行
:param inputs: 輸入
:param training: 默認(rèn)為None
:return: 返回輸出
"""
x = self.fc1(inputs)
x = tf.nn.relu(x)
x = self.fc2(x)
x = tf.nn.relu(x)
x = self.fc3(x)
x = tf.nn.relu(x)
x = self.fc4(x)
x = tf.nn.relu(x)
x = self.fc5(x)
return x
Layer:
class My_Dense(tf.keras.layers.Layer): # 繼承Layer
def __init__(self, input_dim, output_dim):
"""
初始化
:param input_dim:
:param output_dim:
"""
super(My_Dense, self).__init__()
# 添加變量
self.kernel = self.add_variable("w", [input_dim, output_dim]) # 權(quán)重
self.bias = self.add_variable("b", [output_dim]) # 偏置
def call(self, inputs, training=None):
"""
在Layer被調(diào)用的時候執(zhí)行, 計算結(jié)果
:param inputs: 輸入
:param training: 默認(rèn)為None
:return: 返回計算結(jié)果
"""
# y = w * x + b
out = inputs @ self.kernel + self.bias
return out
案例
數(shù)據(jù)集介紹
CIFAR-10 是由 10 類不同的物品組成的 6 萬張彩色圖片的數(shù)據(jù)集. 其中 5 萬張為訓(xùn)練集, 1 萬張為測試集.
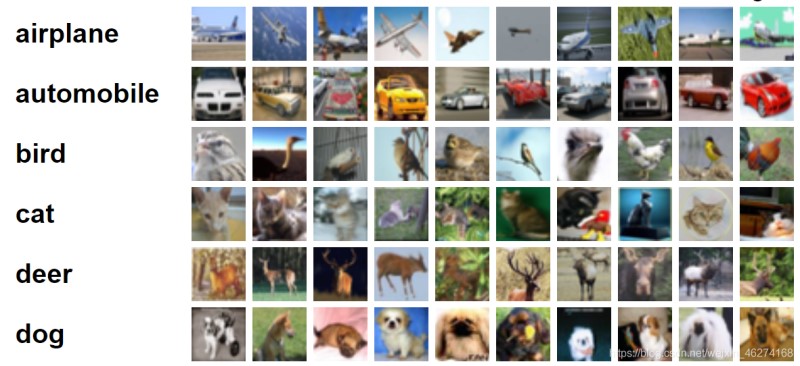
完整代碼
import tensorflow as tf
def pre_process(x, y):
# 轉(zhuǎn)換x
x = 2 * tf.cast(x, dtype=tf.float32) / 255 - 1 # 轉(zhuǎn)換為-1~1的形式
x = tf.reshape(x, [-1, 32 * 32 * 3]) # 把x鋪平
# 轉(zhuǎn)換y
y = tf.convert_to_tensor(y) # 轉(zhuǎn)換為0~1的形式
y = tf.one_hot(y, depth=10) # 轉(zhuǎn)成one_hot編碼
# 返回x, y
return x, y
def get_data():
"""
獲取數(shù)據(jù)
:return:
"""
# 獲取數(shù)據(jù)
(X_train, y_train), (X_test, y_test) = tf.keras.datasets.cifar10.load_data()
# 調(diào)試輸出維度
print(X_train.shape) # (50000, 32, 32, 3)
print(y_train.shape) # (50000, 1)
# squeeze
y_train = tf.squeeze(y_train) # (50000, 1) => (50000,)
y_test = tf.squeeze(y_test) # (10000, 1) => (10000,)
# 分割訓(xùn)練集
train_db = tf.data.Dataset.from_tensor_slices((X_train, y_train)).shuffle(10000, seed=0)
train_db = train_db.batch(batch_size).map(pre_process).repeat(iteration_num) # 迭代20次
# 分割測試集
test_db = tf.data.Dataset.from_tensor_slices((X_test, y_test)).shuffle(10000, seed=0)
test_db = test_db.batch(batch_size).map(pre_process)
return train_db, test_db
class My_Dense(tf.keras.layers.Layer): # 繼承Layer
def __init__(self, input_dim, output_dim):
"""
初始化
:param input_dim:
:param output_dim:
"""
super(My_Dense, self).__init__()
# 添加變量
self.kernel = self.add_weight("w", [input_dim, output_dim]) # 權(quán)重
self.bias = self.add_weight("b", [output_dim]) # 偏置
def call(self, inputs, training=None):
"""
在Layer被調(diào)用的時候執(zhí)行, 計算結(jié)果
:param inputs: 輸入
:param training: 默認(rèn)為None
:return: 返回計算結(jié)果
"""
# y = w * x + b
out = inputs @ self.kernel + self.bias
return out
class My_Model(tf.keras.Model): # 繼承Model
def __init__(self):
"""
初始化
"""
super(My_Model, self).__init__()
self.fc1 = My_Dense(32 * 32 * 3, 256) # 第一層
self.fc2 = My_Dense(256, 128) # 第二層
self.fc3 = My_Dense(128, 64) # 第三層
self.fc4 = My_Dense(64, 32) # 第四層
self.fc5 = My_Dense(32, 10) # 第五層
def call(self, inputs, training=None):
"""
在Model被調(diào)用的時候執(zhí)行
:param inputs: 輸入
:param training: 默認(rèn)為None
:return: 返回輸出
"""
x = self.fc1(inputs)
x = tf.nn.relu(x)
x = self.fc2(x)
x = tf.nn.relu(x)
x = self.fc3(x)
x = tf.nn.relu(x)
x = self.fc4(x)
x = tf.nn.relu(x)
x = self.fc5(x)
return x
# 定義超參數(shù)
batch_size = 256 # 一次訓(xùn)練的樣本數(shù)目
learning_rate = 0.001 # 學(xué)習(xí)率
iteration_num = 20 # 迭代次數(shù)
optimizer = tf.keras.optimizers.Adam(learning_rate=learning_rate) # 優(yōu)化器
loss = tf.losses.CategoricalCrossentropy(from_logits=True) # 損失
network = My_Model() # 實例化網(wǎng)絡(luò)
# 調(diào)試輸出summary
network.build(input_shape=[None, 32 * 32 * 3])
print(network.summary())
# 組合
network.compile(optimizer=optimizer,
loss=loss,
metrics=["accuracy"])
if __name__ == "__main__":
# 獲取分割的數(shù)據(jù)集
train_db, test_db = get_data()
# 擬合
network.fit(train_db, epochs=5, validation_data=test_db, validation_freq=1)
輸出結(jié)果:
Model: "my__model"
_________________________________________________________________
Layer (type) Output Shape Param #
=================================================================
my__dense (My_Dense) multiple 786688
_________________________________________________________________
my__dense_1 (My_Dense) multiple 32896
_________________________________________________________________
my__dense_2 (My_Dense) multiple 8256
_________________________________________________________________
my__dense_3 (My_Dense) multiple 2080
_________________________________________________________________
my__dense_4 (My_Dense) multiple 330
=================================================================
Total params: 830,250
Trainable params: 830,250
Non-trainable params: 0
_________________________________________________________________
None
(50000, 32, 32, 3)
(50000, 1)
2021-06-15 14:35:26.600766: I tensorflow/compiler/mlir/mlir_graph_optimization_pass.cc:176] None of the MLIR Optimization Passes are enabled (registered 2)
Epoch 1/5
3920/3920 [==============================] - 39s 10ms/step - loss: 0.9676 - accuracy: 0.6595 - val_loss: 1.8961 - val_accuracy: 0.5220
Epoch 2/5
3920/3920 [==============================] - 41s 10ms/step - loss: 0.3338 - accuracy: 0.8831 - val_loss: 3.3207 - val_accuracy: 0.5141
Epoch 3/5
3920/3920 [==============================] - 41s 10ms/step - loss: 0.1713 - accuracy: 0.9410 - val_loss: 4.2247 - val_accuracy: 0.5122
Epoch 4/5
3920/3920 [==============================] - 41s 10ms/step - loss: 0.1237 - accuracy: 0.9581 - val_loss: 4.9458 - val_accuracy: 0.5050
Epoch 5/5
3920/3920 [==============================] - 42s 11ms/step - loss: 0.1003 - accuracy: 0.9666 - val_loss: 5.2425 - val_accuracy: 0.5097
到此這篇關(guān)于一小時學(xué)會TensorFlow2之自定義層的文章就介紹到這了,更多相關(guān)TensorFlow2自定義層內(nèi)容請搜索腳本之家以前的文章或繼續(xù)瀏覽下面的相關(guān)文章希望大家以后多多支持腳本之家!
您可能感興趣的文章:- 解決Pytorch自定義層出現(xiàn)多Variable共享內(nèi)存錯誤問題
- 解決Keras 自定義層時遇到版本的問題
- keras Lambda自定義層實現(xiàn)數(shù)據(jù)的切片方式,Lambda傳參數(shù)
- 使用Keras加載含有自定義層或函數(shù)的模型操作